Imagine Alzheimer’s disease (AD) as a mysterious land waiting to be explored. In this exciting study, researchers set out on a quest to discover potential biomarkers for AD. They carefully examined the expression profiles of AD and control tissue samples using four different diagnostic models. By comparing the differences in gene expression, they identified a set of genes called differentially expressed genes (DEGs). These DEGs were then subjected to enrichment analysis to find common pathways associated with AD. Next, the researchers used advanced machine learning algorithms, such as the random forest and logistic regression models, to construct a diagnostic model. After assessing multiple models, they found that the least absolute shrinkage and selection operator (LASSO) model performed the best. This model identified eight feature genes that showed great promise as potential AD biomarkers. To dig deeper into their findings, the researchers also explored how specific miRNAs regulate these genes. Additionally, they investigated the infiltration of immune cells in AD patients using a technique called single-sample gene set enrichment analysis (ssGSEA). The results revealed high levels of dendritic cells and plasmacytoid dendritic cells in AD patients. These findings open up new avenues for the development of innovative strategies for treating individuals with AD. If you’re curious to learn more about this groundbreaking research, dive into the full article!
BackgroundDespite tremendous progress in diagnosis and prediction of Alzheimer’s disease (AD), the absence of treatments implies the need for further research. In this study, we screened AD biomarkers by comparing expression profiles of AD and control tissue samples and used various models to identify potential biomarkers. We further explored immune cells associated with these biomarkers that are involved in the brain microenvironment.MethodsBy differential expression analysis, we identified differentially expressed genes (DEGs) of four datasets (GSE125583, GSE118553, GSE5281, GSE122063), and common expression direction of genes of four datasets were considered as intersecting DEGs, which were used to perform enrichment analysis. We then screened the intersecting pathways between the pathways identified by enrichment analysis. DEGs in intersecting pathways that had an area under the curve (AUC) > 0.7 constructed random forest, least absolute shrinkage and selection operator (LASSO), logistic regression, and gradient boosting machine models. Subsequently, using receiver operating characteristic curve (ROC) and decision curve analysis (DCA) to select an optimal diagnostic model, we obtained the feature genes. Feature genes that were regulated by differentially expressed miRNAs (AUC > 0.85) were explored further. Furthermore, using single-sample GSEA to calculate infiltration of immune cells in AD patients.ResultsScreened 1855 intersecting DEGs that were involved in RAS and AMPK signaling. The LASSO model performed best among the four models. Thus, it was used as the optimal diagnostic model for ROC and DCA analyses. This obtained eight feature genes, including ATP2B3, BDNF, DVL2, ITGA10, SLC6A12, SMAD4, SST, and TPI1. SLC6A12 is regulated by miR-3176. Finally, the results of ssGSEA indicated dendritic cells and plasmacytoid dendritic cells were highly infiltrated in AD patients.ConclusionThe LASSO model is the optimal diagnostic model for identifying feature genes as potential AD biomarkers, which can supply new strategies for the treatment of patients with AD.
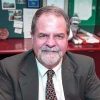
Dr. David Lowemann, M.Sc, Ph.D., is a co-founder of the Institute for the Future of Human Potential, where he leads the charge in pioneering Self-Enhancement Science for the Success of Society. With a keen interest in exploring the untapped potential of the human mind, Dr. Lowemann has dedicated his career to pushing the boundaries of human capabilities and understanding.
Armed with a Master of Science degree and a Ph.D. in his field, Dr. Lowemann has consistently been at the forefront of research and innovation, delving into ways to optimize human performance, cognition, and overall well-being. His work at the Institute revolves around a profound commitment to harnessing cutting-edge science and technology to help individuals lead more fulfilling and intelligent lives.
Dr. Lowemann’s influence extends to the educational platform BetterSmarter.me, where he shares his insights, findings, and personal development strategies with a broader audience. His ongoing mission is shaping the way we perceive and leverage the vast capacities of the human mind, offering invaluable contributions to society’s overall success and collective well-being.