Designing a neural decoding system is like crafting a recipe with many ingredients. One crucial step is finding the right combination of parameters to achieve the desired outcome. Just as a chef adjusts the amount of salt and spices to create the perfect flavor, scientists fine-tune various parameters in real-time neural decoding systems to optimize their performance. However, with numerous parameters to consider, it’s like navigating a vast design space. In this study, researchers propose an innovative optimization framework that automatically configures the parameters in neural decoders, unlocking new levels of accuracy and efficiency. The framework is rigorously evaluated through two case studies, revealing significant improvements over manual parameter optimization methods. To further speed up the process, the researchers also explore multi-threading strategies. By providing an efficient and effective method for parameter estimation, this optimization framework empowers researchers to push the boundaries of neural decoding and experiment with alternative decoding models.
Real-time neuron detection and neural activity extraction are critical components of real-time neural decoding. They are modeled effectively in dataflow graphs. However, these graphs and the components within them in general have many parameters, including hyper-parameters associated with machine learning sub-systems. The dataflow graph parameters induce a complex design space, where alternative configurations (design points) provide different trade-offs involving key operational metrics including accuracy and time-efficiency. In this paper, we propose a novel optimization framework that automatically configures the parameters in different neural decoders. The proposed optimization framework is evaluated in depth through two case studies. Significant performance improvement in terms of accuracy and efficiency is observed in both case studies compared to the manual parameter optimization that was associated with the published results of those case studies. Additionally, we investigate the application of efficient multi-threading strategies to speed-up the running time of our parameter optimization framework. Our proposed optimization framework enables efficient and effective estimation of parameters, which leads to more powerful neural decoding capabilities and allows researchers to experiment more easily with alternative decoding models.
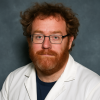
Dr. David Lowemann, M.Sc, Ph.D., is a co-founder of the Institute for the Future of Human Potential, where he leads the charge in pioneering Self-Enhancement Science for the Success of Society. With a keen interest in exploring the untapped potential of the human mind, Dr. Lowemann has dedicated his career to pushing the boundaries of human capabilities and understanding.
Armed with a Master of Science degree and a Ph.D. in his field, Dr. Lowemann has consistently been at the forefront of research and innovation, delving into ways to optimize human performance, cognition, and overall well-being. His work at the Institute revolves around a profound commitment to harnessing cutting-edge science and technology to help individuals lead more fulfilling and intelligent lives.
Dr. Lowemann’s influence extends to the educational platform BetterSmarter.me, where he shares his insights, findings, and personal development strategies with a broader audience. His ongoing mission is shaping the way we perceive and leverage the vast capacities of the human mind, offering invaluable contributions to society’s overall success and collective well-being.