Imagine you’re driving a car and learning a new skill, like parallel parking. But instead of having a driving instructor by your side, you have a computer interface that reads your brain signals to guide you. Brain-computer interfaces (BCIs) have incredible potential, but there’s a problem: many people struggle to produce brain patterns that the computer can understand. To solve this, researchers are developing new training methods that help users control their brain activity more effectively. In this study, scientists propose using Riemannian geometry-based metrics – fancy mathematical tools – to measure and reinforce user performance during BCI training. They tested different variations of these metrics and found that they accurately reflected changes in user performance compared to standard methods. These metrics could be a valuable way to evaluate and track progress in BCI training. The next step is figuring out how to present this feedback to users in a way that helps them improve even more. If you’re interested in the fascinating world of brain-computer interfaces and want to dive deeper, check out the full article!
Despite growing interest and research into brain-computer interfaces (BCI), their usage remains limited outside of research laboratories. One reason for this is BCI inefficiency, the phenomenon where a significant number of potential users are unable to produce machine-discernible brain signal patterns to control the devices. To reduce the prevalence of BCI inefficiency, some have advocated for novel user-training protocols that enable users to more effectively modulate their neural activity. Important considerations for the design of these protocols are the assessment measures that are used for evaluating user performance and for providing feedback that guides skill acquisition. Herein, we present three trial-wise adaptations (running, sliding window and weighted average) of Riemannian geometry-based user-performance metrics (classDistinct reflecting the degree of class separability and classStability reflecting the level of within-class consistency) to enable feedback to the user following each individual trial. We evaluated these metrics, along with conventional classifier feedback, using simulated and previously recorded sensorimotor rhythm-BCI data to assess their correlation with and discrimination of broader trends in user performance. Analysis revealed that the sliding window and weighted average variants of our proposed trial-wise Riemannian geometry-based metrics more accurately reflected performance changes during BCI sessions compared to conventional classifier output. The results indicate the metrics are a viable method for evaluating and tracking user performance changes during BCI-user training and, therefore, further investigation into how these metrics may be presented to users during training is warranted.
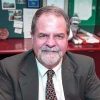
Dr. David Lowemann, M.Sc, Ph.D., is a co-founder of the Institute for the Future of Human Potential, where he leads the charge in pioneering Self-Enhancement Science for the Success of Society. With a keen interest in exploring the untapped potential of the human mind, Dr. Lowemann has dedicated his career to pushing the boundaries of human capabilities and understanding.
Armed with a Master of Science degree and a Ph.D. in his field, Dr. Lowemann has consistently been at the forefront of research and innovation, delving into ways to optimize human performance, cognition, and overall well-being. His work at the Institute revolves around a profound commitment to harnessing cutting-edge science and technology to help individuals lead more fulfilling and intelligent lives.
Dr. Lowemann’s influence extends to the educational platform BetterSmarter.me, where he shares his insights, findings, and personal development strategies with a broader audience. His ongoing mission is shaping the way we perceive and leverage the vast capacities of the human mind, offering invaluable contributions to society’s overall success and collective well-being.