Imagine you’re trying to make a delicious smoothie, but you need to remove the tough outer layer of the fruit first. Similarly, in brain sciences, researchers need to strip away the skull to get a clear view of the brain during MRI analysis. However, existing methods designed for human brains struggle when it comes to non-human primate brains. It’s like trying to slice apples with a knife that’s too dull. To address this challenge, scientists have developed the HC-Net, which is like having a super sharp and versatile knife for primate brain extraction. The HC-Net uses a combination of 3D and 2D convolutions, making use of spatial information between adjacent slices of MRI images to achieve remarkable accuracy without putting too much strain on computational resources. It’s like harnessing the power of both your right and left hands to get the job done efficiently! The HC-Net model has been thoroughly tested on macaque brain data from different sites, demonstrating impressive performance in terms of inference time and accuracy. Achieving a mean Dice coefficient of 95.46%, it outshines other methods in precision. Additionally, the HC-Net showcases its adaptability and reliability across various brain extraction tasks. So if you want to dive deeper into this groundbreaking research and uncover more about how HC-Net transforms primate brain extraction, check out the full article!
Brain extraction (skull stripping) is an essential step in the magnetic resonance imaging (MRI) analysis of brain sciences. However, most of the current brain extraction methods that achieve satisfactory results for human brains are often challenged by non-human primate brains. Due to the small sample characteristics and the nature of thick-slice scanning of macaque MRI data, traditional deep convolutional neural networks (DCNNs) are unable to obtain excellent results. To overcome this challenge, this study proposed a symmetrical end-to-end trainable hybrid convolutional neural network (HC-Net). It makes full use of the spatial information between adjacent slices of the MRI image sequence and combines three consecutive slices from three axes for 3D convolutions, which reduces the calculation consumption and promotes accuracy. The HC-Net consists of encoding and decoding structures of 3D convolutions and 2D convolutions in series. The effective use of 2D convolutions and 3D convolutions relieves the underfitting of 2D convolutions to spatial features and the overfitting of 3D convolutions to small samples. After evaluating macaque brain data from different sites, the results showed that HC-Net performed better in inference time (approximately 13 s per volume) and accuracy (mean Dice coefficient reached 95.46%). The HC-Net model also had good generalization ability and stability in different modes of brain extraction tasks.
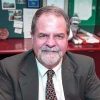
Dr. David Lowemann, M.Sc, Ph.D., is a co-founder of the Institute for the Future of Human Potential, where he leads the charge in pioneering Self-Enhancement Science for the Success of Society. With a keen interest in exploring the untapped potential of the human mind, Dr. Lowemann has dedicated his career to pushing the boundaries of human capabilities and understanding.
Armed with a Master of Science degree and a Ph.D. in his field, Dr. Lowemann has consistently been at the forefront of research and innovation, delving into ways to optimize human performance, cognition, and overall well-being. His work at the Institute revolves around a profound commitment to harnessing cutting-edge science and technology to help individuals lead more fulfilling and intelligent lives.
Dr. Lowemann’s influence extends to the educational platform BetterSmarter.me, where he shares his insights, findings, and personal development strategies with a broader audience. His ongoing mission is shaping the way we perceive and leverage the vast capacities of the human mind, offering invaluable contributions to society’s overall success and collective well-being.