Imagine you have a treasure chest filled with precious gems, each representing a different aspect of Parkinson’s disease. That’s what the Parkinson’s Progression Markers Initiative (PPMI) has at its disposal – over a decade’s worth of diverse and valuable data from patients, control subjects, and those at risk. Just like mining for gems, researchers are using machine learning methods to extract meaningful insights from this immense dataset. In this review, we take a closer look at how machine learning has been applied to analyze the PPMI data. We discover that while there is significant variation in the types of data and models used, there is still untapped potential in fully harnessing the unique features of the PPMI data set. By diving into each dimension – data types, models, and validation procedures – we gain a deeper understanding of the challenges faced and offer recommendations for future machine learning studies in the PPMI cohort. If you’re curious about how applying machine learning can unveil new understandings of Parkinson’s disease and pave the way for more personalized care, grab your gear and embark on the exhilarating adventure of exploring this research!
The Parkinson’s Progression Markers Initiative (PPMI) has collected more than a decade’s worth of longitudinal and multi-modal data from patients, healthy controls, and at-risk individuals, including imaging, clinical, cognitive, and ‘omics’ biospecimens. Such a rich dataset presents unprecedented opportunities for biomarker discovery, patient subtyping, and prognostic prediction, but it also poses challenges that may require the development of novel methodological approaches to solve. In this review, we provide an overview of the application of machine learning methods to analyzing data from the PPMI cohort. We find that there is significant variability in the types of data, models, and validation procedures used across studies, and that much of what makes the PPMI data set unique (multi-modal and longitudinal observations) remains underutilized in most machine learning studies. We review each of these dimensions in detail and provide recommendations for future machine learning work using data from the PPMI cohort.
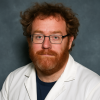
Dr. David Lowemann, M.Sc, Ph.D., is a co-founder of the Institute for the Future of Human Potential, where he leads the charge in pioneering Self-Enhancement Science for the Success of Society. With a keen interest in exploring the untapped potential of the human mind, Dr. Lowemann has dedicated his career to pushing the boundaries of human capabilities and understanding.
Armed with a Master of Science degree and a Ph.D. in his field, Dr. Lowemann has consistently been at the forefront of research and innovation, delving into ways to optimize human performance, cognition, and overall well-being. His work at the Institute revolves around a profound commitment to harnessing cutting-edge science and technology to help individuals lead more fulfilling and intelligent lives.
Dr. Lowemann’s influence extends to the educational platform BetterSmarter.me, where he shares his insights, findings, and personal development strategies with a broader audience. His ongoing mission is shaping the way we perceive and leverage the vast capacities of the human mind, offering invaluable contributions to society’s overall success and collective well-being.