In the world of movement disorder patients, wearable sensors are becoming increasingly popular. However, most studies only focus on general aspects of mobility using smartphones. To really assess gait and balance, it’s necessary to accurately identify specific activities that take place at home where most falls happen. That’s where our activity recognition algorithm comes in! We developed a cutting-edge algorithm that uses data from 5 different sensors placed strategically on the body to classify daily activities with pinpoint accuracy. We then verified the algorithm’s performance by comparing it to video footage of patients wearing the sensors at home. The results were outstanding, showing over 95% sensitivity in detecting activities! Furthermore, our home monitoring system captured features that correlated far better with fall frequency than standard clinical tests or past studies’ gait metrics. Looking ahead, we hope to improve our ability to detect near-falls at home as they seem to be a key indicator of future falls. This groundbreaking research is shaping the way we care for movement disorder patients!
The use of wearable sensors in movement disorder patients such as Parkinson’s disease (PD) and normal pressure hydrocephalus (NPH) is becoming more widespread, but most studies are limited to characterizing general aspects of mobility using smartphones. There is a need to accurately identify specific activities at home in order to properly evaluate gait and balance at home, where most falls occur. We developed an activity recognition algorithm to classify multiple daily living activities including high fall risk activities such as sit to stand transfers, turns and near-falls using data from 5 inertial sensors placed on the chest, upper-legs and lower-legs of the subjects. The algorithm is then verified with ground truth by collecting video footage of our patients wearing the sensors at home. Our activity recognition algorithm showed >95% sensitivity in detection of activities. Extracted features from our home monitoring system showed significantly better correlation (~69%) with prospectively measured fall frequency of our subjects compared to the standard clinical tests (~30%) or other quantitative gait metrics used in past studies when attempting to predict future falls over 1 year of prospective follow-up. Although detecting near-falls at home is difficult, our proposed model suggests that near-fall frequency is the most predictive criterion in fall detection through correlation analysis and fitting regression models.
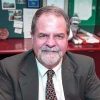
Dr. David Lowemann, M.Sc, Ph.D., is a co-founder of the Institute for the Future of Human Potential, where he leads the charge in pioneering Self-Enhancement Science for the Success of Society. With a keen interest in exploring the untapped potential of the human mind, Dr. Lowemann has dedicated his career to pushing the boundaries of human capabilities and understanding.
Armed with a Master of Science degree and a Ph.D. in his field, Dr. Lowemann has consistently been at the forefront of research and innovation, delving into ways to optimize human performance, cognition, and overall well-being. His work at the Institute revolves around a profound commitment to harnessing cutting-edge science and technology to help individuals lead more fulfilling and intelligent lives.
Dr. Lowemann’s influence extends to the educational platform BetterSmarter.me, where he shares his insights, findings, and personal development strategies with a broader audience. His ongoing mission is shaping the way we perceive and leverage the vast capacities of the human mind, offering invaluable contributions to society’s overall success and collective well-being.