Imagine trying to crack a secret code that reveals the hidden workings of your brain! Well, that’s exactly what scientists are doing with the help of machine learning. By analyzing brain MRI data and cognitive test scores, researchers have developed models that can predict an individual’s cognitive performance and detect patterns associated with mild cognitive impairment (MCI) and Alzheimer’s disease. They found that as we age, our cognitive functioning may slightly change, but it generally remains stable. However, in people with MCI or dementia, there is a converging decline in performance around 100 years of age. Using these machine learning models, researchers were able to accurately classify participants into three groups: cognitively normal elderly individuals, those with MCI, and those with dementia. The most accurate model relied on voxel-based morphometry data to predict cognitive scores. By decoding these patterns, scientists hope to gain a deeper understanding of brain aging and develop improved diagnostic tools for neurodegenerative diseases. To learn more about this fascinating research, check out the full article!
Cracking the Code of Cognitive Decline: Predicting Alzheimer’s with Brain-Machine Learning
BackgroundThe combined analysis of imaging and functional modalities is supposed to improve diagnostics of neurodegenerative diseases with advanced data science techniques.ObjectiveTo get an insight into normal and accelerated brain aging by developing the machine learning models that predict individual performance in neuropsychological and cognitive tests from brain MRI. With these models we endeavor to look for patterns of brain structure-function association (SFA) indicative of mild cognitive impairment (MCI) and Alzheimer’s dementia.Materials and methodsWe explored the age-related variability of cognitive and neuropsychological test scores in normal and accelerated aging and constructed regression models predicting functional performance in cognitive tests from brain radiomics data. The models were trained on the three study cohorts from ADNI dataset—cognitively normal individuals, patients with MCI or dementia—separately. We also looked for significant correlations between cortical parcellation volumes and test scores in the cohorts to investigate neuroanatomical differences in relation to cognitive status. Finally, we worked out an approach for the classification of the examinees according to the pattern of structure-function associations into the cohorts of the cognitively normal elderly and patients with MCI or dementia.ResultsIn the healthy population, the global cognitive functioning slightly changes with age. It also remains stable across the disease course in the majority of cases. In healthy adults and patients with MCI or dementia, the trendlines of performance in digit symbol substitution test and trail making test converge at the approximated point of 100 years of age. According to the SFA pattern, we distinguish three cohorts: the cognitively normal elderly, patients with MCI, and dementia. The highest accuracy is achieved with the model trained to predict the mini-mental state examination score from voxel-based morphometry data. The application of the majority voting technique to models predicting results in cognitive tests improved the classification performance up to 91.95% true positive rate for healthy participants, 86.21%—for MCI and 80.18%—for dementia cases.ConclusionThe machine learning model, when trained on the cases of this of that group, describes a disease-specific SFA pattern. The pattern serves as a “stamp” of the disease reflected by the model.
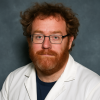
Dr. David Lowemann, M.Sc, Ph.D., is a co-founder of the Institute for the Future of Human Potential, where he leads the charge in pioneering Self-Enhancement Science for the Success of Society. With a keen interest in exploring the untapped potential of the human mind, Dr. Lowemann has dedicated his career to pushing the boundaries of human capabilities and understanding.
Armed with a Master of Science degree and a Ph.D. in his field, Dr. Lowemann has consistently been at the forefront of research and innovation, delving into ways to optimize human performance, cognition, and overall well-being. His work at the Institute revolves around a profound commitment to harnessing cutting-edge science and technology to help individuals lead more fulfilling and intelligent lives.
Dr. Lowemann’s influence extends to the educational platform BetterSmarter.me, where he shares his insights, findings, and personal development strategies with a broader audience. His ongoing mission is shaping the way we perceive and leverage the vast capacities of the human mind, offering invaluable contributions to society’s overall success and collective well-being.