Imagine you have a mold and you’re trying to create a very specific shape. Now, imagine trying to make that shape without ever touching the mold directly. That’s what researchers are doing in the field of Parkinson’s disease (PD) assessment. Currently, the standard method for evaluating motor symptoms in PD involves physical contact between trained examiners and patients. But what if there was a way to assess rigidity and postural stability remotely? This study aimed to develop scoring models for these factors using a low-cost RGB algorithm and other motion data extracted from the Movement Disorder Society’s Unified Parkinson’s Disease Rating Scale Part III (MDS-UPDRS III) evaluation. The results showed promising levels of accuracy and correlation for the models, demonstrating their potential for remote assessments in situations where social distancing is necessary, such as during the COVID-19 pandemic. If you’re curious to learn more about this innovative approach to PD assessment, check out the full article!
Background and objectivesThe Movement Disorder Society’s Unified Parkinson’s Disease Rating Scale Part III (MDS-UPDRS III) is mostly common used for assessing the motor symptoms of Parkinson’s disease (PD). In remote circumstances, vision-based techniques have many strengths over wearable sensors. However, rigidity (item 3.3) and postural stability (item 3.12) in the MDS-UPDRS III cannot be assessed remotely since participants need to be touched by a trained examiner during testing. We developed the four scoring models of rigidity of the neck, rigidity of the lower extremities, rigidity of the upper extremities, and postural stability based on features extracted from other available and touchless motions.MethodsThe red, green, and blue (RGB) computer vision algorithm and machine learning were combined with other available motions from the MDS-UPDRS III evaluation. A total of 104 patients with PD were split into a train set (89 individuals) and a test set (15 individuals). The light gradient boosting machine (LightGBM) multiclassification model was trained. Weighted kappa (k), absolute accuracy (ACC ± 0), and Spearman’s correlation coefficient (rho) were used to evaluate the performance of model.ResultsFor model of rigidity of the upper extremities, k = 0.58 (moderate), ACC ± 0 = 0.73, and rho = 0.64 (moderate). For model of rigidity of the lower extremities, k = 0.66 (substantial), ACC ± 0 = 0.70, and rho = 0.76 (strong). For model of rigidity of the neck, k = 0.60 (moderate), ACC ± 0 = 0.73, and rho = 0.60 (moderate). For model of postural stability, k = 0.66 (substantial), ACC ± 0 = 0.73, and rho = 0.68 (moderate).ConclusionOur study can be meaningful for remote assessments, especially when people have to maintain social distance, e.g., in situations such as the coronavirus disease-2019 (COVID-19) pandemic.
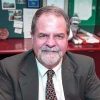
Dr. David Lowemann, M.Sc, Ph.D., is a co-founder of the Institute for the Future of Human Potential, where he leads the charge in pioneering Self-Enhancement Science for the Success of Society. With a keen interest in exploring the untapped potential of the human mind, Dr. Lowemann has dedicated his career to pushing the boundaries of human capabilities and understanding.
Armed with a Master of Science degree and a Ph.D. in his field, Dr. Lowemann has consistently been at the forefront of research and innovation, delving into ways to optimize human performance, cognition, and overall well-being. His work at the Institute revolves around a profound commitment to harnessing cutting-edge science and technology to help individuals lead more fulfilling and intelligent lives.
Dr. Lowemann’s influence extends to the educational platform BetterSmarter.me, where he shares his insights, findings, and personal development strategies with a broader audience. His ongoing mission is shaping the way we perceive and leverage the vast capacities of the human mind, offering invaluable contributions to society’s overall success and collective well-being.