Imagine a cluttered room filled with toys scattered everywhere. To navigate through this chaotic scene, unmanned systems need to be able to detect not only the objects but also the best way to grasp them. This is no easy task! But fear not, scientists have developed a clever neural learning approach called SOGD that can predict the ideal grasp configuration for each detected object. It’s like having a robot friend who knows exactly how to pick up every toy in the room! By filtering out the cluttered background and training two separate branches to detect objects and grasp candidates, SOGD learns the relationship between them using an alignment module. The results of experiments on real-world datasets show that SOGD outperforms other state-of-the-art methods in predicting reasonable grasp configurations from messy scenes.
Object detection and grasp detection are essential for unmanned systems working in cluttered real-world environments. Detecting grasp configurations for each object in the scene would enable reasoning manipulations. However, finding the relationships between objects and grasp configurations is still a challenging problem. To achieve this, we propose a novel neural learning approach, namely SOGD, to predict a best grasp configuration for each detected objects from an RGB-D image. The cluttered background is first filtered out via a 3D-plane-based approach. Then two separate branches are designed to detect objects and grasp candidates, respectively. The relationship between object proposals and grasp candidates are learned by an additional alignment module. A series of experiments are conducted on two public datasets (Cornell Grasp Dataset and Jacquard Dataset) and the results demonstrate the superior performance of our SOGD against SOTA methods in predicting reasonable grasp configurations “from a cluttered scene.”
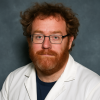
Dr. David Lowemann, M.Sc, Ph.D., is a co-founder of the Institute for the Future of Human Potential, where he leads the charge in pioneering Self-Enhancement Science for the Success of Society. With a keen interest in exploring the untapped potential of the human mind, Dr. Lowemann has dedicated his career to pushing the boundaries of human capabilities and understanding.
Armed with a Master of Science degree and a Ph.D. in his field, Dr. Lowemann has consistently been at the forefront of research and innovation, delving into ways to optimize human performance, cognition, and overall well-being. His work at the Institute revolves around a profound commitment to harnessing cutting-edge science and technology to help individuals lead more fulfilling and intelligent lives.
Dr. Lowemann’s influence extends to the educational platform BetterSmarter.me, where he shares his insights, findings, and personal development strategies with a broader audience. His ongoing mission is shaping the way we perceive and leverage the vast capacities of the human mind, offering invaluable contributions to society’s overall success and collective well-being.