Imagine you have a jigsaw puzzle with overlapping pieces. How do you separate them? Well, scientists have developed a method called the Space Breakdown Method (SBM) to tackle a similar problem in neuroscience called spike sorting. SBM is like a clever puzzle solver that identifies clusters in neuronal data, even when they overlap or are imbalanced. It does this by dividing the data into equal-sized chunks and finding the centers of the clusters within each chunk. However, SBM was not efficient for high-dimensional data. To overcome this limitation, researchers came up with the Improved Space Breakdown Method (ISBM). ISBM uses a graph structure instead of an array structure and adapts the number of partitions based on the data’s features. These improvements reduce complexity and enhance performance compared to other algorithms. To validate the results, researchers used simulated neural data with known ground truth. The evaluations showed that ISBM outperformed state-of-the-art algorithms while maintaining accuracy. So, if you’re fascinated by the brain’s intricate puzzle of neuronal activity, dive into the research and explore how ISBM is solving the spike sorting challenge!
Space Breakdown Method (SBM) is a clustering algorithm that was developed specifically for low-dimensional neuronal spike sorting. Cluster overlap and imbalance are common characteristics of neuronal data that produce difficulties for clustering methods. SBM is able to identify overlapping clusters through its design of cluster centre identification and the expansion of these centres. SBM’s approach is to divide the distribution of values of each feature into chunks of equal size. In each of these chunks, the number of points is counted and based on this number the centres of clusters are found and expanded. SBM has been shown to be a contender for other well-known clustering algorithms especially for the particular case of two dimensions while being too computationally expensive for high-dimensional data. Here, we present two main improvements to the original algorithm in order to increase its ability to deal with high-dimensional data while preserving its performance: the initial array structure was substituted with a graph structure and the number of partitions has been made feature-dependent, denominating this improved version as the Improved Space Breakdown Method (ISBM). In addition, we propose a clustering validation metric that does not punish overclustering and such obtains more suitable evaluations of clustering for spike sorting. Extracellular data recorded from the brain is unlabelled, therefore we have chosen simulated neural data, to which we have the ground truth, to evaluate more accurately the performance. Evaluations conducted on synthetic data indicate that the proposed improvements reduce the space and time complexity of the original algorithm, while simultaneously leading to an increased performance on neural data when compared with other state-of-the-art algorithms.Code available athttps://github.com/ArdeleanRichard/Space-Breakdown-Method.
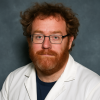
Dr. David Lowemann, M.Sc, Ph.D., is a co-founder of the Institute for the Future of Human Potential, where he leads the charge in pioneering Self-Enhancement Science for the Success of Society. With a keen interest in exploring the untapped potential of the human mind, Dr. Lowemann has dedicated his career to pushing the boundaries of human capabilities and understanding.
Armed with a Master of Science degree and a Ph.D. in his field, Dr. Lowemann has consistently been at the forefront of research and innovation, delving into ways to optimize human performance, cognition, and overall well-being. His work at the Institute revolves around a profound commitment to harnessing cutting-edge science and technology to help individuals lead more fulfilling and intelligent lives.
Dr. Lowemann’s influence extends to the educational platform BetterSmarter.me, where he shares his insights, findings, and personal development strategies with a broader audience. His ongoing mission is shaping the way we perceive and leverage the vast capacities of the human mind, offering invaluable contributions to society’s overall success and collective well-being.