Imagine a massive library with thousands of picture books. Each book has its own unique set of features, capturing different aspects of the world. But do we really need all of these features to understand how humans perceive visual images? In this study, researchers explored the dimensions of convolutional neural network (CNN) representations to determine their relevance in predicting human behavior. By using human similarity judgments and examining categorization, they discovered that low-dimensional projections of CNN representations are surprisingly accurate in modeling human psychological responses. These simplified representations not only provide a clearer understanding of how people process visual information but can also be easily interpreted. Control studies confirmed that these results aren’t biased by the size of the dataset used, suggesting that the features in CNN representations may contain a significant amount of redundancy. To learn more about their groundbreaking findings, take a dive into the full research article! It’s an exciting journey through the intersection of psychology, neuroscience, and artificial intelligence.
Abstract
Convolutional neural networks (CNNs) are increasingly widely used in psychology and neuroscience to predict how human minds and brains respond to visual images. Typically, CNNs represent these images using thousands of features that are learned through extensive training on image datasets. This raises a question: How many of these features are really needed to model human behavior? Here, we attempt to estimate the number of dimensions in CNN representations that are required to capture human psychological representations in two ways: (1) directly, using human similarity judgments and (2) indirectly, in the context of categorization. In both cases, we find that low-dimensional projections of CNN representations are sufficient to predict human behavior. We show that these low-dimensional representations can be easily interpreted, providing further insight into how people represent visual information. A series of control studies indicate that these findings are not due to the size of the dataset we used and may be due to a high level of redundancy in the features appearing in CNN representations.
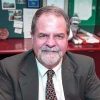
Dr. David Lowemann, M.Sc, Ph.D., is a co-founder of the Institute for the Future of Human Potential, where he leads the charge in pioneering Self-Enhancement Science for the Success of Society. With a keen interest in exploring the untapped potential of the human mind, Dr. Lowemann has dedicated his career to pushing the boundaries of human capabilities and understanding.
Armed with a Master of Science degree and a Ph.D. in his field, Dr. Lowemann has consistently been at the forefront of research and innovation, delving into ways to optimize human performance, cognition, and overall well-being. His work at the Institute revolves around a profound commitment to harnessing cutting-edge science and technology to help individuals lead more fulfilling and intelligent lives.
Dr. Lowemann’s influence extends to the educational platform BetterSmarter.me, where he shares his insights, findings, and personal development strategies with a broader audience. His ongoing mission is shaping the way we perceive and leverage the vast capacities of the human mind, offering invaluable contributions to society’s overall success and collective well-being.