Deep learning is like a magician that has wowed us with its incredible abilities in computer tasks. But here’s the thing — this illusionist needs a big bag of tricks, or in this case, a large training dataset, to perform its best. Unfortunately, in real-world applications, it’s not always easy to get our hands on abundant samples. That’s where few-shot learning steps in. It’s like teaching a magic trick to someone using just a handful of props and their prior knowledge. In this mini-review, we zoom in on few-shot classification and explore recent methods that make the most out of limited samples. We start by defining the few-shot classification problem and then present a newly organized taxonomy. We dive deep into different application scenarios, weigh the pros and cons of various methods, and categorize them into four perspectives: data augmentation, metric-based method, optimization method, and model-based method. By the end, we wrap things up with a promising conclusion and discuss what the future holds for unlocking the true potential of few samples in deep learning.
Deep learning has achieved enormous success in various computer tasks. The excellent performance depends heavily on adequate training datasets, however, it is difficult to obtain abundant samples in practical applications. Few-shot learning is proposed to address the data limitation problem in the training process, which can perform rapid learning with few samples by utilizing prior knowledge. In this paper, we focus on few-shot classification to conduct a survey about the recent methods. First, we elaborate on the definition of the few-shot classification problem. Then we propose a newly organized taxonomy, discuss the application scenarios in which each method is effective, and compare the pros and cons of different methods. We classify few-shot image classification methods from four perspectives: (i) Data augmentation, which contains sample-level and task-level data augmentation. (ii) Metric-based method, which analyzes both feature embedding and metric function. (iii) Optimization method, which is compared from the aspects of self-learning and mutual learning. (iv) Model-based method, which is discussed from the perspectives of memory-based, rapid adaptation and multi-task learning. Finally, we conduct the conclusion and prospect of this paper.
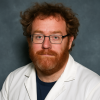
Dr. David Lowemann, M.Sc, Ph.D., is a co-founder of the Institute for the Future of Human Potential, where he leads the charge in pioneering Self-Enhancement Science for the Success of Society. With a keen interest in exploring the untapped potential of the human mind, Dr. Lowemann has dedicated his career to pushing the boundaries of human capabilities and understanding.
Armed with a Master of Science degree and a Ph.D. in his field, Dr. Lowemann has consistently been at the forefront of research and innovation, delving into ways to optimize human performance, cognition, and overall well-being. His work at the Institute revolves around a profound commitment to harnessing cutting-edge science and technology to help individuals lead more fulfilling and intelligent lives.
Dr. Lowemann’s influence extends to the educational platform BetterSmarter.me, where he shares his insights, findings, and personal development strategies with a broader audience. His ongoing mission is shaping the way we perceive and leverage the vast capacities of the human mind, offering invaluable contributions to society’s overall success and collective well-being.