Imagine your cardiovascular system as a treasure chest filled with clues about your age. Well, that’s exactly what researchers discovered! They used machine learning on various measures of cardiovascular function to estimate participants’ calendar age. Just like a skilled detective piecing together evidence, the researchers analyzed data from electrocardiograms and blood pressure recordings of healthy volunteers. By considering factors like heart rate variability, blood pressure variability, and pulse wave dynamics, they built models to accurately predict age. The best model, called Gaussian process regression (GPR), had an impressive correlation of 0.81 and an average error of only 5.6 years! Interestingly, the estimates were slightly more accurate for men than women. But when it came to obese participants, the models tended to overestimate their age, hinting at advanced cardiovascular aging. This research opens up exciting possibilities for using these estimated ages as markers of cardiovascular health. Imagine a simple measurement that can provide valuable insights into your heart’s well-being! Future studies will explore whether these estimates can also predict the risk of developing heart problems. Ready to dive deeper into this fascinating science? Check out the full article for more details!
IntroductionAging is accompanied by physiological changes in cardiovascular regulation that can be evaluated using a variety of metrics. In this study, we employ machine learning on autonomic cardiovascular indices in order to estimate participants’ age.MethodsWe analyzed a database including resting state electrocardiogram and continuous blood pressure recordings of healthy volunteers. A total of 884 data sets met the inclusion criteria. Data of 72 other participants with an BMI indicating obesity (>30 kg/m²) were withheld as an evaluation sample. For all participants, 29 different cardiovascular indices were calculated including heart rate variability, blood pressure variability, baroreflex function, pulse wave dynamics, and QT interval characteristics. Based on cardiovascular indices, sex and device, four different approaches were applied in order to estimate the calendar age of healthy subjects, i.e., relevance vector regression (RVR), Gaussian process regression (GPR), support vector regression (SVR), and linear regression (LR). To estimate age in the obese group, we drew normal-weight controls from the large sample to build a training set and a validation set that had an age distribution similar to the obesity test sample.ResultsIn a five-fold cross validation scheme, we found the GPR model to be suited best to estimate calendar age, with a correlation of r=0.81 and a mean absolute error of MAE=5.6 years. In men, the error (MAE=5.4 years) seemed to be lower than that in women (MAE=6.0 years). In comparison to normal-weight subjects, GPR and SVR significantly overestimated the age of obese participants compared with controls. The highest age gap indicated advanced cardiovascular aging by 5.7 years in obese participants.DiscussionIn conclusion, machine learning can be used to estimate age on cardiovascular function in a healthy population when considering previous models of biological aging. The estimated age might serve as a comprehensive and readily interpretable marker of cardiovascular function. Whether it is a useful risk predictor should be investigated in future studies.
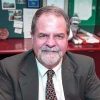
Dr. David Lowemann, M.Sc, Ph.D., is a co-founder of the Institute for the Future of Human Potential, where he leads the charge in pioneering Self-Enhancement Science for the Success of Society. With a keen interest in exploring the untapped potential of the human mind, Dr. Lowemann has dedicated his career to pushing the boundaries of human capabilities and understanding.
Armed with a Master of Science degree and a Ph.D. in his field, Dr. Lowemann has consistently been at the forefront of research and innovation, delving into ways to optimize human performance, cognition, and overall well-being. His work at the Institute revolves around a profound commitment to harnessing cutting-edge science and technology to help individuals lead more fulfilling and intelligent lives.
Dr. Lowemann’s influence extends to the educational platform BetterSmarter.me, where he shares his insights, findings, and personal development strategies with a broader audience. His ongoing mission is shaping the way we perceive and leverage the vast capacities of the human mind, offering invaluable contributions to society’s overall success and collective well-being.