Imagine a bustling city filled with scientists and innovators, all working tirelessly to unravel the mysteries of the human brain. In this vibrant metropolis, one particular area of research has recently taken center stage: brain-computer interfaces (BCI). Think of BCI as a futuristic translator that allows our brains to communicate directly with computers, opening up a world of possibilities for neurorehabilitation and brain injury patients. Over the years, scientists have developed various methods to harness the power of electroencephalogram (EEG) data in BCI applications. In the past, these methods relied heavily on matrix factorization and machine learning due to limited EEG datasets. However, thanks to recent advancements, deep learning has emerged as a game-changer in the field. Using large, high-quality EEG datasets, researchers are now employing deep learning models to tackle complex tasks like motor imagery classification and epileptic seizure detection. The potential is immense, and this study dives deep into the promises and challenges of deep learning-based approaches for EEG-based BCIs. It highlights the merits and drawbacks of these models while pointing towards future directions for research. If you’re curious about how deep learning can revolutionize the way we interact with our brains, dive into this fascinating study!
In the previous decade, breakthroughs in the central nervous system bioinformatics and computational innovation have prompted significant developments in brain–computer interface (BCI), elevating it to the forefront of applied science and research. BCI revitalization enables neurorehabilitation strategies for physically disabled patients (e.g., disabled patients and hemiplegia) and patients with brain injury (e.g., patients with stroke). Different methods have been developed for electroencephalogram (EEG)-based BCI applications. Due to the lack of a large set of EEG data, methods using matrix factorization and machine learning were the most popular. However, things have changed recently because a number of large, high-quality EEG datasets are now being made public and used in deep learning-based BCI applications. On the other hand, deep learning is demonstrating great prospects for solving complex relevant tasks such as motor imagery classification, epileptic seizure detection, and driver attention recognition using EEG data. Researchers are doing a lot of work on deep learning-based approaches in the BCI field right now. Moreover, there is a great demand for a study that emphasizes only deep learning models for EEG-based BCI applications. Therefore, we introduce this study to the recent proposed deep learning-based approaches in BCI using EEG data (from 2017 to 2022). The main differences, such as merits, drawbacks, and applications are introduced. Furthermore, we point out current challenges and the directions for future studies. We argue that this review study will help the EEG research community in their future research.
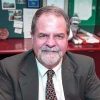
Dr. David Lowemann, M.Sc, Ph.D., is a co-founder of the Institute for the Future of Human Potential, where he leads the charge in pioneering Self-Enhancement Science for the Success of Society. With a keen interest in exploring the untapped potential of the human mind, Dr. Lowemann has dedicated his career to pushing the boundaries of human capabilities and understanding.
Armed with a Master of Science degree and a Ph.D. in his field, Dr. Lowemann has consistently been at the forefront of research and innovation, delving into ways to optimize human performance, cognition, and overall well-being. His work at the Institute revolves around a profound commitment to harnessing cutting-edge science and technology to help individuals lead more fulfilling and intelligent lives.
Dr. Lowemann’s influence extends to the educational platform BetterSmarter.me, where he shares his insights, findings, and personal development strategies with a broader audience. His ongoing mission is shaping the way we perceive and leverage the vast capacities of the human mind, offering invaluable contributions to society’s overall success and collective well-being.