Imagine you have a friend who loves solving puzzles. One day, they show you a special puzzle that can predict whether someone has a skin condition called atopic dermatitis (AD). Instead of relying on subjective judgment, this puzzle uses a combination of boosted particle swarm optimization (PSO) and fuzzy k-nearest neighbor (FKNN) to make accurate predictions! The boosted PSO algorithm ensures that the puzzle pieces are evenly distributed, improving the accuracy of the predictions. Additionally, the FKNN classifier is optimized using binary SRWPSO to enhance its classification performance. By solving this puzzle, the key features of AD can be identified, such as lymphocyte content, presence of cat dander, and levels of allergens like milk and ragweed. This innovative bSRWPSO-FKNN method brings us one step closer to effective diagnosis and management of AD!
IntroductionAtopic dermatitis (AD) is an allergic disease with extreme itching that bothers patients. However, diagnosing AD depends on clinicians’ subjective judgment, which may be missed or misdiagnosed sometimes.MethodsThis paper establishes a medical prediction model for the first time on the basis of the enhanced particle swarm optimization (SRWPSO) algorithm and the fuzzy K-nearest neighbor (FKNN), called bSRWPSO-FKNN, which is practiced on a dataset related to patients with AD. In SRWPSO, the Sobol sequence is introduced into particle swarm optimization (PSO) to make the particle distribution of the initial population more uniform, thus improving the population’s diversity and traversal. At the same time, this study also adds a random replacement strategy and adaptive weight strategy to the population updating process of PSO to overcome the shortcomings of poor convergence accuracy and easily fall into the local optimum of PSO. In bSRWPSO-FKNN, the core of which is to optimize the classification performance of FKNN through binary SRWPSO.ResultsTo prove that the study has scientific significance, this paper first successfully demonstrates the core advantages of SRWPSO in well-known algorithms through benchmark function validation experiments. Secondly, this article demonstrates that the bSRWPSO-FKNN has practical medical significance and effectiveness through nine public and medical datasets.DiscussionThe 10 times 10-fold cross-validation experiments demonstrate that bSRWPSO-FKNN can pick up the key features of AD, including the content of lymphocytes (LY), Cat dander, Milk, Dermatophagoides Pteronyssinus/Farinae, Ragweed, Cod, and Total IgE. Therefore, the established bSRWPSO-FKNN method practically aids in the diagnosis of AD.
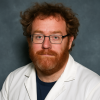
Dr. David Lowemann, M.Sc, Ph.D., is a co-founder of the Institute for the Future of Human Potential, where he leads the charge in pioneering Self-Enhancement Science for the Success of Society. With a keen interest in exploring the untapped potential of the human mind, Dr. Lowemann has dedicated his career to pushing the boundaries of human capabilities and understanding.
Armed with a Master of Science degree and a Ph.D. in his field, Dr. Lowemann has consistently been at the forefront of research and innovation, delving into ways to optimize human performance, cognition, and overall well-being. His work at the Institute revolves around a profound commitment to harnessing cutting-edge science and technology to help individuals lead more fulfilling and intelligent lives.
Dr. Lowemann’s influence extends to the educational platform BetterSmarter.me, where he shares his insights, findings, and personal development strategies with a broader audience. His ongoing mission is shaping the way we perceive and leverage the vast capacities of the human mind, offering invaluable contributions to society’s overall success and collective well-being.