Imagine your brain as a bustling city, with different neighborhoods responsible for different functions. One important neighborhood, called the default mode network (DMN), plays a crucial role in cognitive processes. In a recent study, scientists investigated how the DMN is connected to regions involved in visual processing and its potential as a biomarker for delayed neurocognitive recovery (DNR). They used resting-state functional magnetic resonance imaging (fMRI) to study 74 patients before surgery and found that DNR patients had significantly decreased connectivity between the DMN and visual processing regions. This suggests that disrupted communication between these brain areas could be a sign of impaired cognitive recovery. To further explore this, the researchers employed powerful machine-learning algorithms to predict DNR based on the functional connectivity features. Their best-performing model, using the random forest algorithm, achieved an impressive accuracy of 84.0% and provided valuable insights into the neural mechanisms of DNR. The study opens up new avenues for understanding how visual processing regions influence cognitive recovery and may help identify individuals at risk for delayed neurocognitive recovery. For more details, dive into the full research article!
ObjectivesThe abnormal functional connectivity (FC) pattern of default mode network (DMN) may be key markers for early identification of various cognitive disorders. However, the whole-brain FC changes of DMN in delayed neurocognitive recovery (DNR) are still unclear. Our study was aimed at exploring the whole-brain FC patterns of all regions in DMN and the potential features as biomarkers for the prediction of DNR using machine-learning algorithms.MethodsResting-state functional magnetic resonance imaging (fMRI) was conducted before surgery on 74 patients undergoing non-cardiac surgery. Seed-based whole-brain FC with 18 core regions located in the DMN was performed, and FC features that were statistically different between the DNR and non-DNR patients after false discovery correction were extracted. Afterward, based on the extracted FC features, machine-learning algorithms such as support vector machine, logistic regression, decision tree, and random forest were established to recognize DNR. The machine learning experiment procedure mainly included three following steps: feature standardization, parameter adjustment, and performance comparison. Finally, independent testing was conducted to validate the established prediction model. The algorithm performance was evaluated by a permutation test.ResultsWe found significantly decreased DMN connectivity with the brain regions involved in visual processing in DNR patients than in non-DNR patients. The best result was obtained from the random forest algorithm based on the 20 decision trees (estimators). The random forest model achieved the accuracy, sensitivity, and specificity of 84.0, 63.1, and 89.5%, respectively. The area under the receiver operating characteristic curve of the classifier reached 86.4%. The feature that contributed the most to the random forest model was the FC between the left retrosplenial cortex/posterior cingulate cortex and left precuneus.ConclusionThe decreased FC of DMN with regions involved in visual processing might be effective markers for the prediction of DNR and could provide new insights into the neural mechanisms of DNR.Clinical Trial Registration: Chinese Clinical Trial Registry, ChiCTR-DCD-15006096.
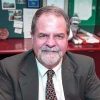
Dr. David Lowemann, M.Sc, Ph.D., is a co-founder of the Institute for the Future of Human Potential, where he leads the charge in pioneering Self-Enhancement Science for the Success of Society. With a keen interest in exploring the untapped potential of the human mind, Dr. Lowemann has dedicated his career to pushing the boundaries of human capabilities and understanding.
Armed with a Master of Science degree and a Ph.D. in his field, Dr. Lowemann has consistently been at the forefront of research and innovation, delving into ways to optimize human performance, cognition, and overall well-being. His work at the Institute revolves around a profound commitment to harnessing cutting-edge science and technology to help individuals lead more fulfilling and intelligent lives.
Dr. Lowemann’s influence extends to the educational platform BetterSmarter.me, where he shares his insights, findings, and personal development strategies with a broader audience. His ongoing mission is shaping the way we perceive and leverage the vast capacities of the human mind, offering invaluable contributions to society’s overall success and collective well-being.