Imagine you’re trying to solve a puzzle. You’re given a sequence of codes, like a secret message, but the catch is that the codes are organized in a hierarchical structure. In this study, researchers wanted to see if people could learn and anticipate the patterns in these sequences, even with minimal information. They used a special grammar called the Fibonacci grammar, which creates unique but similar sequences. By analyzing participants’ response times, they found that people were not simply relying on detecting repeated patterns or statistical probabilities. Instead, they were able to understand and predict upcoming codes based on their understanding of the hierarchy. In fact, participants showed sensitivity to the nested structure of the sequences, indicating that they were able to mentally organize the information into smaller embedded sections. The researchers believe that this ability to recognize and merge hierarchical transitions is what allowed participants to successfully learn and anticipate the patterns within the binary sequences. To dive deeper into the fascinating world of hierarchical learning in binary sequences, check out the full article!
Hierarchical Learning in Binary Sequences through Fibonacci Grammar
Abstract
In this article, we explore the extraction of recursive nested structure in the processing of binary sequences. Our aim was to determine whether humans learn the higher-order regularities of a highly simplified input where only sequential-order information marks the hierarchical structure. To this end, we implemented a sequence generated by the Fibonacci grammar in a serial reaction time task. This deterministic grammar generates aperiodic but self-similar sequences. The combination of these two properties allowed us to evaluate hierarchical learning while controlling for the use of low-level strategies like detecting recurring patterns. The deterministic aspect of the grammar allowed us to predict precisely which points in the sequence should be subject to anticipation. Results showed that participants’ pattern of anticipation could not be accounted for by “flat” statistical learning processes and was consistent with them anticipating upcoming points based on hierarchical assumptions. We also found that participants were sensitive to the structure constituency, suggesting that they organized the signal into embedded constituents. We hypothesized that the participants built this structure by merging recursively deterministic transitions.
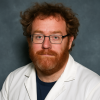
Dr. David Lowemann, M.Sc, Ph.D., is a co-founder of the Institute for the Future of Human Potential, where he leads the charge in pioneering Self-Enhancement Science for the Success of Society. With a keen interest in exploring the untapped potential of the human mind, Dr. Lowemann has dedicated his career to pushing the boundaries of human capabilities and understanding.
Armed with a Master of Science degree and a Ph.D. in his field, Dr. Lowemann has consistently been at the forefront of research and innovation, delving into ways to optimize human performance, cognition, and overall well-being. His work at the Institute revolves around a profound commitment to harnessing cutting-edge science and technology to help individuals lead more fulfilling and intelligent lives.
Dr. Lowemann’s influence extends to the educational platform BetterSmarter.me, where he shares his insights, findings, and personal development strategies with a broader audience. His ongoing mission is shaping the way we perceive and leverage the vast capacities of the human mind, offering invaluable contributions to society’s overall success and collective well-being.