Imagine if our brains were a symphony, and artificial neural networks were the conductors! In this study, researchers delve into the intricate world of cognitive science by using artificial neural networks (ANNs) to uncover how the mind perceives and represents musical properties. By training ANNs to classify musical chords, they uncover fascinating insights into the underlying structure of cognition. Astonishingly, they discover that the connection weights between input units and hidden units can be described using Fourier phase spaces, a concept from musical set theory. These weighted connections carry a measure of similarity between two Fourier structures: the hidden unit’s weights and the stimulus structure. Even though hidden units do not explicitly compute these Fourier structures, output units use this similarity measure to classify chords. Notably, different types of units with distinct activation functions utilize this measure in different ways. The results challenge traditional notions of Fourier analysis in musical set theory while revealing that Fourier representations are not solely confined to this domain of music theory. These findings have far-reaching implications for cognitive psychologists exploring the role of Fourier representations in musical cognition. To fully appreciate these intriguing discoveries, dive into the research!
Abstract
How might artificial neural networks (ANNs) inform cognitive science? Often cognitive scientists use ANNs but do not examine their internal structures. In this paper, we use ANNs to explore how cognition might represent musical properties. We train ANNs to classify musical chords, and we interpret network structure to determine what representations ANNs discover and use. We find connection weights between input units and hidden units can be described using Fourier phase spaces, a representation studied in musical set theory. We find the total signal coming through these weighted connection weights is a measure of the similarity between two Fourier structures: the structure of the hidden unit’s weights and the structure of the stimulus. This is surprising because neither of these Fourier structures is computed by the hidden unit. We then show how output units use such similarity measures to classify chords. However, we also find different types of units—units that use different activation functions—use this similarity measure very differently. This result, combined with other findings, indicates that while our networks are related to the Fourier analysis of musical sets, they do not perform Fourier analyses of the kind usually described in musical set theory. Our results show Fourier representations of music are not limited to musical set theory. Our results also suggest how cognitive psychologists might explore Fourier representations in musical cognition. Critically, such theoretical and empirical implications require researchers to understand how network structure converts stimuli into responses.
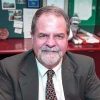
Dr. David Lowemann, M.Sc, Ph.D., is a co-founder of the Institute for the Future of Human Potential, where he leads the charge in pioneering Self-Enhancement Science for the Success of Society. With a keen interest in exploring the untapped potential of the human mind, Dr. Lowemann has dedicated his career to pushing the boundaries of human capabilities and understanding.
Armed with a Master of Science degree and a Ph.D. in his field, Dr. Lowemann has consistently been at the forefront of research and innovation, delving into ways to optimize human performance, cognition, and overall well-being. His work at the Institute revolves around a profound commitment to harnessing cutting-edge science and technology to help individuals lead more fulfilling and intelligent lives.
Dr. Lowemann’s influence extends to the educational platform BetterSmarter.me, where he shares his insights, findings, and personal development strategies with a broader audience. His ongoing mission is shaping the way we perceive and leverage the vast capacities of the human mind, offering invaluable contributions to society’s overall success and collective well-being.