Imagine trying to unlock the secrets of a complex code, where the meaning of each expression can be encoded in strict truth conditions. But here’s the twist – language is often vague, and everyone interprets it differently. In this study, researchers set out to understand how people understand vague concepts by using a computational model of decision-making. They focused on two quantifiers, ‘most’ and ‘more than half,’ and conducted online experiments with native English speakers. By analyzing participants’ verification tasks, they discovered that there were significant differences in how individuals represented the meaning of ‘most.’ They also found that the verification process for ‘most’ relies on proportions, as indicated by reaction times and model parameters. Additionally, the researchers explored whether these representations remained stable over time and found that they did. These findings challenge existing semantic theories and contribute to our understanding of how we represent vague concepts. This study opens up exciting possibilities for further research in experimental linguistics!
Cracking the Code of Language with Decision-Making
Abstract
According to logical theories of meaning, a meaning of an expression can be formalized and encoded in truth conditions. Vagueness of the language and individual differences between people are a challenge to incorporate into the meaning representations. In this paper, we propose a new approach to study truth-conditional representations of vague concepts. For a case study, we selected two natural language quantifiers most and more than half. We conducted two online experiments, each with 90 native English speakers. In the first experiment, we tested between-subjects variability in meaning representations. In the second experiment, we tested the stability of meaning representations over time by testing the same group of participants in two experimental sessions. In both experiments, participants performed the verification task. They verified a sentence with a quantifier (e.g., “Most of the gleerbs are feezda.”) based on the numerical information provided in the second sentence, (e.g., “60% of the gleerbs are feezda”). To investigate between-subject and within-subject differences in meaning representations, we proposed an extended version of the Diffusion Decision Model with two parameters capturing truth conditions and vagueness. We fit the model to responses and reaction times data. In the first experiment, we found substantial between-subject differences in representations of most as reflected by the variability in the truth conditions. Moreover, we found that the verification of most is proportion-dependent as reflected in the reaction time effect and model parameter. In the second experiment, we showed that quantifier representations are stable over time as reflected in stable model parameters across two experimental sessions. These findings challenge semantic theories that assume the truth-conditional equivalence of most and more than half and contribute to the representational theory of vague concepts. The current study presents a promising approach to study semantic representations, which can have a wide application in experimental linguistics.
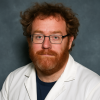
Dr. David Lowemann, M.Sc, Ph.D., is a co-founder of the Institute for the Future of Human Potential, where he leads the charge in pioneering Self-Enhancement Science for the Success of Society. With a keen interest in exploring the untapped potential of the human mind, Dr. Lowemann has dedicated his career to pushing the boundaries of human capabilities and understanding.
Armed with a Master of Science degree and a Ph.D. in his field, Dr. Lowemann has consistently been at the forefront of research and innovation, delving into ways to optimize human performance, cognition, and overall well-being. His work at the Institute revolves around a profound commitment to harnessing cutting-edge science and technology to help individuals lead more fulfilling and intelligent lives.
Dr. Lowemann’s influence extends to the educational platform BetterSmarter.me, where he shares his insights, findings, and personal development strategies with a broader audience. His ongoing mission is shaping the way we perceive and leverage the vast capacities of the human mind, offering invaluable contributions to society’s overall success and collective well-being.