Imagine you’re trying to pass a secret message down a line of people. The way you connect with each person and the properties of their bodies can greatly influence how well the message gets across. In the same way, information transmission in networks, both biological and artificial, relies on connectivity between units and the characteristics of those units. But scientists have been puzzled about how certain biophysical parameters, like the membrane properties of neurons and the delays between action potentials, affect this transmission. A recent study delved into these parameters using a spiking neural network as a model. They found that different parameter values had a significant impact on metrics of information transfer, such as coherence and classification accuracy. Interestingly, they discovered that higher firing rates were crucial for accurate information transmission with leaky integrate-and-fire units, while adaptive integrate-and-fire units showed lower firing rates but still achieved high information transmission. These findings shed light on how the brain’s cells may transfer information and have implications for the predictive coding theory of the cerebral cortex. To dive deeper into this fascinating research, check out the full article.
IntroductionInformation transmission and representation in both natural and artificial networks is dependent on connectivity between units. Biological neurons, in addition, modulate synaptic dynamics and post-synaptic membrane properties, but how these relate to information transmission in a population of neurons is still poorly understood. A recent study investigated local learning rules and showed how a spiking neural network can learn to represent continuous signals. Our study builds on their model to explore how basic membrane properties and synaptic delays affect information transfer.MethodsThe system consisted of three input and output units and a hidden layer of 300 excitatory and 75 inhibitory leaky integrate-and-fire (LIF) or adaptive integrate-and-fire (AdEx) units. After optimizing the connectivity to accurately replicate the input patterns in the output units, we transformed the model to more biologically accurate units and included synaptic delay and concurrent action potential generation in distinct neurons. We examined three different parameter regimes which comprised either identical physiological values for both excitatory and inhibitory units (Comrade), more biologically accurate values (Bacon), or the Comrade regime whose output units were optimized for low reconstruction error (HiFi). We evaluated information transmission and classification accuracy of the network with four distinct metrics: coherence, Granger causality, transfer entropy, and reconstruction error.ResultsBiophysical parameters showed a major impact on information transfer metrics. The classification was surprisingly robust, surviving very low firing and information rates, whereas information transmission overall and particularly low reconstruction error were more dependent on higher firing rates in LIF units. In AdEx units, the firing rates were lower and less information was transferred, but interestingly the highest information transmission rates were no longer overlapping with the highest firing rates.DiscussionOur findings can be reflected on the predictive coding theory of the cerebral cortex and may suggest information transfer qualities as a phenomenological quality of biological cells.
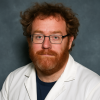
Dr. David Lowemann, M.Sc, Ph.D., is a co-founder of the Institute for the Future of Human Potential, where he leads the charge in pioneering Self-Enhancement Science for the Success of Society. With a keen interest in exploring the untapped potential of the human mind, Dr. Lowemann has dedicated his career to pushing the boundaries of human capabilities and understanding.
Armed with a Master of Science degree and a Ph.D. in his field, Dr. Lowemann has consistently been at the forefront of research and innovation, delving into ways to optimize human performance, cognition, and overall well-being. His work at the Institute revolves around a profound commitment to harnessing cutting-edge science and technology to help individuals lead more fulfilling and intelligent lives.
Dr. Lowemann’s influence extends to the educational platform BetterSmarter.me, where he shares his insights, findings, and personal development strategies with a broader audience. His ongoing mission is shaping the way we perceive and leverage the vast capacities of the human mind, offering invaluable contributions to society’s overall success and collective well-being.