When it comes to detecting and segmenting brain metastases, relying on manual methods can be time-consuming and labor-intensive. However, a new study has found that deep learning models, specifically 2.5D and 3D convolution neural networks, can greatly improve this process. The models were trained and tested on multinational MRI data from two independent centers, using the dice similarity coefficient and detection sensitivity as evaluation metrics. The results showed that both the 2.5D and 3D models achieved similar segmentation performance, with the former demonstrating better detection rate and the latter producing fewer false positives. Moreover, a comparative benchmark called nnU-Net performed even better in terms of minimizing false positives. These findings indicate the potential of deep learning in accurately segmenting brain metastases with minimal errors. However, it is worth noting that the accuracy of the models decreases for metastases smaller than 0.4 cm2. Overall, this research highlights the benefits of deep learning in improving brain metastases segmentation and encourages further exploration into its applications in medical imaging.
IntroductionManagement of patients with brain metastases is often based on manual lesion detection and segmentation by an expert reader. This is a time- and labor-intensive process, and to that end, this work proposes an end-to-end deep learning segmentation network for a varying number of available MRI available sequences.MethodsWe adapt and evaluate a 2.5D and a 3D convolution neural network trained and tested on a retrospective multinational study from two independent centers, in addition, nnU-Net was adapted as a comparative benchmark. Segmentation and detection performance was evaluated by: (1) the dice similarity coefficient, (2) a per-metastases and the average detection sensitivity, and (3) the number of false positives.ResultsThe 2.5D and 3D models achieved similar results, albeit the 2.5D model had better detection rate, whereas the 3D model had fewer false positive predictions, and nnU-Net had fewest false positives, but with the lowest detection rate. On MRI data from center 1, the 2.5D, 3D, and nnU-Net detected 79%, 71%, and 65% of all metastases; had an average per patient sensitivity of 0.88, 0.84, and 0.76; and had on average 6.2, 3.2, and 1.7 false positive predictions per patient, respectively. For center 2, the 2.5D, 3D, and nnU-Net detected 88%, 86%, and 78% of all metastases; had an average per patient sensitivity of 0.92, 0.91, and 0.85; and had on average 1.0, 0.4, and 0.1 false positive predictions per patient, respectively.Discussion/ConclusionOur results show that deep learning can yield highly accurate segmentations of brain metastases with few false positives in multinational data, but the accuracy degrades for metastases with an area smaller than 0.4 cm2.
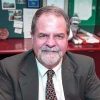
Dr. David Lowemann, M.Sc, Ph.D., is a co-founder of the Institute for the Future of Human Potential, where he leads the charge in pioneering Self-Enhancement Science for the Success of Society. With a keen interest in exploring the untapped potential of the human mind, Dr. Lowemann has dedicated his career to pushing the boundaries of human capabilities and understanding.
Armed with a Master of Science degree and a Ph.D. in his field, Dr. Lowemann has consistently been at the forefront of research and innovation, delving into ways to optimize human performance, cognition, and overall well-being. His work at the Institute revolves around a profound commitment to harnessing cutting-edge science and technology to help individuals lead more fulfilling and intelligent lives.
Dr. Lowemann’s influence extends to the educational platform BetterSmarter.me, where he shares his insights, findings, and personal development strategies with a broader audience. His ongoing mission is shaping the way we perceive and leverage the vast capacities of the human mind, offering invaluable contributions to society’s overall success and collective well-being.