Just like a synchronized dance requires harmony between dancers, our brain’s ability to imagine movements relies on the coordinated interaction between different regions. This study delves into the contribution of these instantaneous interactions between electrodes in classifying motor imagery tasks, specifically left-hand and right-hand movements. By using correlation and Jaccard distance as measures, two classifier models (linear discriminant analysis and hidden conditional random field) were analyzed. The study also explored the impact of using the sliding window technique in these models. The results? Adding temporal features significantly improved the classification accuracy for both models, showcasing the importance of this dynamic information. Additionally, interactions between electrodes played a crucial role in enhancing classifier performance, regardless of the interaction measure or classifier type used. Curious about how our brains perform this mesmerizing dance of thoughts? Explore the full article for an inside look!
The purpose of this study is to analyze the contribution of the interactions between electrodes, measured either as correlation or as Jaccard distance, to the classification of two actions in a motor imagery paradigm, namely, left-hand movement and right-hand movement. The analysis is performed in two classifier models, namely, a static (linear discriminant analysis, LDA) model and a dynamic (hidden conditional random field, HCRF) model. The impact of using the sliding window technique (SWT) in the static and dynamic models is also analyzed. The study proved that their combination with temporal features provides significant information to improve the classification in a two-class motor imagery task for LDA (average accuracy: 0.7192 no additional features, 0.7617 by adding correlation, 0.7606 by adding Jaccard distance; p < 0.001) and HCRF (average accuracy: 0.7370 no additional features, 0.7764 by adding correlation, 0.7793 by adding Jaccard distance; p < 0.001). Also, we showed that adding interactions between electrodes improves significantly the performance of each classifier, regarding the nature of the interaction measure or the classifier itself.
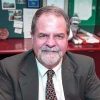
Dr. David Lowemann, M.Sc, Ph.D., is a co-founder of the Institute for the Future of Human Potential, where he leads the charge in pioneering Self-Enhancement Science for the Success of Society. With a keen interest in exploring the untapped potential of the human mind, Dr. Lowemann has dedicated his career to pushing the boundaries of human capabilities and understanding.
Armed with a Master of Science degree and a Ph.D. in his field, Dr. Lowemann has consistently been at the forefront of research and innovation, delving into ways to optimize human performance, cognition, and overall well-being. His work at the Institute revolves around a profound commitment to harnessing cutting-edge science and technology to help individuals lead more fulfilling and intelligent lives.
Dr. Lowemann’s influence extends to the educational platform BetterSmarter.me, where he shares his insights, findings, and personal development strategies with a broader audience. His ongoing mission is shaping the way we perceive and leverage the vast capacities of the human mind, offering invaluable contributions to society’s overall success and collective well-being.