Imagine a group of brilliant scientists in Wenzhou, China who have created a magnificent machine learning model that can predict the severity prognosis in patients with pulmonary embolism (PE). Just like a swarm of intelligent bees working together, this innovative algorithm, called SSACS-KELM, combines the power of the salp swarm algorithm (SSA) and cuckoo search (CS) to recognize and discriminate the severity of PE using patient’s basic information and serum biomarkers. Through extensive experiments and comparisons, they found that indicators like high D-dimer concentration and hypoalbuminemia are crucial for diagnosing PE. The results? A staggering 99.33% accuracy in predicting the severity of PE! This breakthrough could revolutionize how doctors diagnose and treat PE, potentially saving countless lives. Curious to know more? Dive into the fascinating research behind this new intelligence in healthcare!
IntroductionPulmonary embolism (PE) is a common thrombotic disease and potentially deadly cardiovascular disorder. The ratio of clinical misdiagnosis and missed diagnosis of PE is very large because patients with PE are asymptomatic or non-specific.MethodsUsing the clinical data from the First Affiliated Hospital of Wenzhou Medical University (Wenzhou, China), we proposed a swarm intelligence algorithm-based kernel extreme learning machine model (SSACS-KELM) to recognize and discriminate the severity of the PE by patient’s basic information and serum biomarkers. First, an enhanced method (SSACS) is presented by combining the salp swarm algorithm (SSA) with the cuckoo search (CS). Then, the SSACS algorithm is introduced into the KELM classifier to propose the SSACS-KELM model to improve the accuracy and stability of the traditional classifier.ResultsIn the experiments, the benchmark optimization performance of SSACS is confirmed by comparing SSACS with five original classical methods and five high-performance improved algorithms through benchmark function experiments. Then, the overall adaptability and accuracy of the SSACS-KELM model are tested using eight public data sets. Further, to highlight the superiority of SSACS-KELM on PE datasets, this paper conducts comparison experiments with other classical classifiers, swarm intelligence algorithms, and feature selection approaches.DiscussionThe experimental results show that high D-dimer concentration, hypoalbuminemia, and other indicators are important for the diagnosis of PE. The classification results showed that the accuracy of the prediction model was 99.33%. It is expected to be a new and accurate method to distinguish the severity of PE.
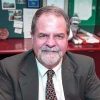
Dr. David Lowemann, M.Sc, Ph.D., is a co-founder of the Institute for the Future of Human Potential, where he leads the charge in pioneering Self-Enhancement Science for the Success of Society. With a keen interest in exploring the untapped potential of the human mind, Dr. Lowemann has dedicated his career to pushing the boundaries of human capabilities and understanding.
Armed with a Master of Science degree and a Ph.D. in his field, Dr. Lowemann has consistently been at the forefront of research and innovation, delving into ways to optimize human performance, cognition, and overall well-being. His work at the Institute revolves around a profound commitment to harnessing cutting-edge science and technology to help individuals lead more fulfilling and intelligent lives.
Dr. Lowemann’s influence extends to the educational platform BetterSmarter.me, where he shares his insights, findings, and personal development strategies with a broader audience. His ongoing mission is shaping the way we perceive and leverage the vast capacities of the human mind, offering invaluable contributions to society’s overall success and collective well-being.