Imagine trying to paint a picture with multiple colored lights shining on your canvas. It’s difficult to accurately perceive the true colors of the objects you’re painting when different light sources create a complex mixture of hues. In the world of computer vision, scientists face a similar challenge when trying to develop color constancy methods that can accurately estimate the true colors of objects in different lighting conditions. One common assumption in these methods is that the light sources have a uniform spectral distribution. However, this assumption often fails when there are multiple light sources with different spectral distributions. A team of researchers has proposed a novel solution called CN-DMS4, which stands for Cascade Network with Deep Multi-Scale Supervision and Single-Scale Estimation. This network takes into account the presence of multiple illuminations by training its parameters in a step-by-step manner, from coarse to fine. By doing so, it aims to estimate the illumination of a scene accurately. The researchers also designed a new loss function to optimize the network’s parameters and reduce the influence of color channels on the estimation process. Experimental results on benchmark datasets showed that their method performs better than previous techniques in terms of both quantitative measurements and visual effects. This study opens up exciting possibilities for future research in multi-illumination estimation in computer vision.
Color constancy methods are generally based on a simplifying assumption that the spectral distribution of a light source is uniform across scenes. However, in reality, this assumption is often violated because of the presence of multiple light sources, that is, more than two illuminations. In this paper, we propose a unique cascade network of deep multi-scale supervision and single-scale estimation (CN-DMS4) to estimate multi-illumination. The network parameters are supervised and learned from coarse to fine in the training process and estimate only the final thinnest level illumination map in the illumination estimation process. Furthermore, to reduce the influence of the color channel on the Euclidean distance or the pixel-level angle error, a new loss function with a channel penalty term is designed to optimize the network parameters. Extensive experiments are conducted on single and multi-illumination benchmark datasets. In comparison with previous multi-illumination estimation methods, our proposed method displays a partial improvement in terms of quantitative data and visual effect, which provides the future research direction in end-to-end multi-illumination estimation.
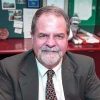
Dr. David Lowemann, M.Sc, Ph.D., is a co-founder of the Institute for the Future of Human Potential, where he leads the charge in pioneering Self-Enhancement Science for the Success of Society. With a keen interest in exploring the untapped potential of the human mind, Dr. Lowemann has dedicated his career to pushing the boundaries of human capabilities and understanding.
Armed with a Master of Science degree and a Ph.D. in his field, Dr. Lowemann has consistently been at the forefront of research and innovation, delving into ways to optimize human performance, cognition, and overall well-being. His work at the Institute revolves around a profound commitment to harnessing cutting-edge science and technology to help individuals lead more fulfilling and intelligent lives.
Dr. Lowemann’s influence extends to the educational platform BetterSmarter.me, where he shares his insights, findings, and personal development strategies with a broader audience. His ongoing mission is shaping the way we perceive and leverage the vast capacities of the human mind, offering invaluable contributions to society’s overall success and collective well-being.