Imagine you’re trying to understand a complex web of relationships. You can tackle it two ways: by studying each piece in isolation or by considering the connections between them. The same principle applies to multi-dimensional time-series (MTS) prediction. Traditional models treat each dimension independently, losing the inter-relationship and vital information. But fear not! A team of scientists has developed a groundbreaking solution: GA-LSTNet, a network based on geometric algebra (GA), which captures the inherent structures and correlations among dimensions. By using GA multi-vectors, they can holistically process MTS without sacrificing the inter-relationships. This approach elevates LSTM and back-propagation through time to the GA domain. The researchers put GA-LSTNet to the test against six other methods using four renowned MTS datasets. With unmatched prediction accuracy, GA-LSTNet outperforms traditional models, triumphantly addressing the shortcomings in MTS prediction. Ready to dive deeper into this revolutionary breakthrough? Read the full article now!
Recent RNN models deal with various dimensions of MTS as independent channels, which may lead to the loss of dependencies between different dimensions or the loss of associated information between each dimension and the global. To process MTS in a holistic way without losing the inter-relationship among dimensions, this paper proposes a novel Long-and Short-term Time-series network based on geometric algebra (GA), dubbed GA-LSTNet. Specifically, taking advantage of GA, multi-dimensional data at each time point of MTS is represented as GA multi-vectors to capture the inherent structures and preserve the correlation of those dimensions. In particular, traditional real-valued RNN, real-valued LSTM, and the back-propagation through time are extended to the GA domain. We evaluate the performance of the proposed GA-LSTNet model in prediction tasks on four well-known MTS datasets, and compared the prediction performance with other six methods. The experimental results indicate that our GA-LSTNet model outperforms traditional real-valued LSTNet with higher prediction accuracy, providing a more accurate solution for the existing shortcomings of MTS prediction models.
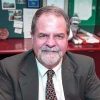
Dr. David Lowemann, M.Sc, Ph.D., is a co-founder of the Institute for the Future of Human Potential, where he leads the charge in pioneering Self-Enhancement Science for the Success of Society. With a keen interest in exploring the untapped potential of the human mind, Dr. Lowemann has dedicated his career to pushing the boundaries of human capabilities and understanding.
Armed with a Master of Science degree and a Ph.D. in his field, Dr. Lowemann has consistently been at the forefront of research and innovation, delving into ways to optimize human performance, cognition, and overall well-being. His work at the Institute revolves around a profound commitment to harnessing cutting-edge science and technology to help individuals lead more fulfilling and intelligent lives.
Dr. Lowemann’s influence extends to the educational platform BetterSmarter.me, where he shares his insights, findings, and personal development strategies with a broader audience. His ongoing mission is shaping the way we perceive and leverage the vast capacities of the human mind, offering invaluable contributions to society’s overall success and collective well-being.