Imagine a heart-pounding race where runners are classified based on their risk of collapsing. In the world of pulmonary embolism (PE), a life-threatening condition, risk classification is crucial for modifying treatment. In a recent study, researchers gathered data from 139 patients, including clinical characteristics and blood analysis. They then developed a prediction framework using machine learning technology. To improve accuracy, the researchers enhanced an algorithm called cuckoo search algorithm (CS) by adding sobol sequence and black hole mechanism. This enhanced algorithm, called SBCS, when combined with kernel extreme learning machine (KELM), showed promising results in diagnosing PE severity. The experiment findings revealed that key indicators such as syncope, systolic blood pressure (SBP), oxygen saturation (SaO2%), white blood cell (WBC) count, and neutrophil percentage (NEUT%) were crucial in assessing the severity of PE. With a remarkable accuracy of 99.26% and sensitivity of 98.57%, this prediction model has the potential to become an accurate and reliable method for distinguishing the severity of PE. Curious to learn more? Dive into the details and explore the underlying research!
IntroductionPulmonary embolism (PE) is a cardiopulmonary condition that can be fatal. PE can lead to sudden cardiovascular collapse and is potentially life-threatening, necessitating risk classification to modify therapy following the diagnosis of PE. We collected clinical characteristics, routine blood data, and arterial blood gas analysis data from all 139 patients.MethodsCombining these data, this paper proposes a PE risk stratified prediction framework based on machine learning technology. An improved algorithm is proposed by adding sobol sequence and black hole mechanism to the cuckoo search algorithm (CS), called SBCS. Based on the coupling of the enhanced algorithm and the kernel extreme learning machine (KELM), a prediction framework is also proposed.ResultsTo confirm the overall performance of SBCS, we run benchmark function experiments in this work. The results demonstrate that SBCS has great convergence accuracy and speed. Then, tests based on seven open data sets are carried out in this study to verify the performance of SBCS on the feature selection problem. To further demonstrate the usefulness and applicability of the SBCS-KELM framework, this paper conducts aided diagnosis experiments on PE data collected from the hospital.DiscussionThe experiment findings show that the indicators chosen, such as syncope, systolic blood pressure (SBP), oxygen saturation (SaO2%), white blood cell (WBC), neutrophil percentage (NEUT%), and others, are crucial for the feature selection approach presented in this study to assess the severity of PE. The classification results reveal that the prediction model’s accuracy is 99.26% and its sensitivity is 98.57%. It is expected to become a new and accurate method to distinguish the severity of PE.
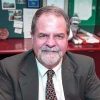
Dr. David Lowemann, M.Sc, Ph.D., is a co-founder of the Institute for the Future of Human Potential, where he leads the charge in pioneering Self-Enhancement Science for the Success of Society. With a keen interest in exploring the untapped potential of the human mind, Dr. Lowemann has dedicated his career to pushing the boundaries of human capabilities and understanding.
Armed with a Master of Science degree and a Ph.D. in his field, Dr. Lowemann has consistently been at the forefront of research and innovation, delving into ways to optimize human performance, cognition, and overall well-being. His work at the Institute revolves around a profound commitment to harnessing cutting-edge science and technology to help individuals lead more fulfilling and intelligent lives.
Dr. Lowemann’s influence extends to the educational platform BetterSmarter.me, where he shares his insights, findings, and personal development strategies with a broader audience. His ongoing mission is shaping the way we perceive and leverage the vast capacities of the human mind, offering invaluable contributions to society’s overall success and collective well-being.