Imagine being able to predict someone’s age just by looking at their brain waves! A group of scientists has developed a new method using deep convolutional neural networks, a type of artificial intelligence, to predict age based on scalp EEG signals. Normally, brain age prediction is done using imaging techniques like MRI, but this study sought to determine if EEG, a much less expensive and more accessible technology, could achieve similar results. The researchers used the TD-BRAIN dataset, which included recordings from over a thousand individuals with and without psychiatric disorders. By training the deep learning model using cross-validation and applying data augmentation techniques to increase the diversity of the training set, they were able to accurately predict age with an error of 5.96 years. Interestingly, they found that the best results were obtained when analyzing both eyes-open and eyes-closed states simultaneously. The frontocentral electrodes were particularly important for age prediction. This research has the potential to provide valuable insights into brain diseases and may serve as a biomarker for diagnosing and assessing various conditions. If you’re interested in learning more about how deep learning is revolutionizing neuroscience, check out the full article!
IntroductionBrain age prediction has been shown to be clinically relevant, with errors in its prediction associated with various psychiatric and neurological conditions. While the prediction from structural and functional magnetic resonance imaging data has been feasible with high accuracy, whether the same results can be achieved with electroencephalography is unclear.MethodsThe current study aimed to create a new deep learning solution for brain age prediction using raw resting-state scalp EEG. To this end, we utilized the TD-BRAIN dataset, including 1,274 subjects (both healthy controls and individuals with various psychiatric disorders, with a total of 1,335 recording sessions). To achieve the best age prediction, we used data augmentation techniques to increase the diversity of the training set and developed a deep convolutional neural network model.ResultsThe model’s training was done with 10-fold cross-subject cross-validation, with the EEG recordings of the subjects used for training not considered to test the model. In training, using the relative rather than the absolute loss function led to a better mean absolute error of 5.96 years in cross-validation. We found that the best performance could be achieved when both eyes-open and eyes-closed states are used simultaneously. The frontocentral electrodes played the most important role in age prediction.DiscussionThe architecture and training method of the proposed deep convolutional neural networks (DCNN) improve state-of-the-art metrics in the age prediction task using raw resting-state EEG data by 13%. Given that brain age prediction might be a potential biomarker of numerous brain diseases, inexpensive and precise EEG-based estimation of brain age will be in demand for clinical practice.
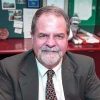
Dr. David Lowemann, M.Sc, Ph.D., is a co-founder of the Institute for the Future of Human Potential, where he leads the charge in pioneering Self-Enhancement Science for the Success of Society. With a keen interest in exploring the untapped potential of the human mind, Dr. Lowemann has dedicated his career to pushing the boundaries of human capabilities and understanding.
Armed with a Master of Science degree and a Ph.D. in his field, Dr. Lowemann has consistently been at the forefront of research and innovation, delving into ways to optimize human performance, cognition, and overall well-being. His work at the Institute revolves around a profound commitment to harnessing cutting-edge science and technology to help individuals lead more fulfilling and intelligent lives.
Dr. Lowemann’s influence extends to the educational platform BetterSmarter.me, where he shares his insights, findings, and personal development strategies with a broader audience. His ongoing mission is shaping the way we perceive and leverage the vast capacities of the human mind, offering invaluable contributions to society’s overall success and collective well-being.