Imagine two puzzle pieces that fit together perfectly, even though they were made by completely different manufacturers. That’s what researchers have discovered when comparing artificial neural networks and the human brain’s auditory system in the field of speech recognition. In a groundbreaking study, scientists utilized advanced technology to map the representations of speech in both artificial and brain neural networks. Astonishingly, they found that the organization of hidden layers in artificial neural networks closely resembled the functional organization of human auditory cortex. Moreover, they were able to improve word recognition accuracy in artificial neural networks by modifying their hidden layer organization based on brain activation patterns. This discovery reveals a remarkable convergence between machines and humans in their ability to understand and process speech. To delve deeper into this exciting research and uncover how artificial and brain neural networks can inform each other, check out the full article!
IntroductionIn recent years, machines powered by deep learning have achieved near-human levels of performance in speech recognition. The fields of artificial intelligence and cognitive neuroscience have finally reached a similar level of performance, despite their huge differences in implementation, and so deep learning models can—in principle—serve as candidates for mechanistic models of the human auditory system.MethodsUtilizing high-performance automatic speech recognition systems, and advanced non-invasive human neuroimaging technology such as magnetoencephalography and multivariate pattern-information analysis, the current study aimed to relate machine-learned representations of speech to recorded human brain representations of the same speech.ResultsIn one direction, we found a quasi-hierarchical functional organization in human auditory cortex qualitatively matched with the hidden layers of deep artificial neural networks trained as part of an automatic speech recognizer. In the reverse direction, we modified the hidden layer organization of the artificial neural network based on neural activation patterns in human brains. The result was a substantial improvement in word recognition accuracy and learned speech representations.DiscussionWe have demonstrated that artificial and brain neural networks can be mutually informative in the domain of speech recognition.
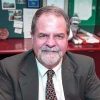
Dr. David Lowemann, M.Sc, Ph.D., is a co-founder of the Institute for the Future of Human Potential, where he leads the charge in pioneering Self-Enhancement Science for the Success of Society. With a keen interest in exploring the untapped potential of the human mind, Dr. Lowemann has dedicated his career to pushing the boundaries of human capabilities and understanding.
Armed with a Master of Science degree and a Ph.D. in his field, Dr. Lowemann has consistently been at the forefront of research and innovation, delving into ways to optimize human performance, cognition, and overall well-being. His work at the Institute revolves around a profound commitment to harnessing cutting-edge science and technology to help individuals lead more fulfilling and intelligent lives.
Dr. Lowemann’s influence extends to the educational platform BetterSmarter.me, where he shares his insights, findings, and personal development strategies with a broader audience. His ongoing mission is shaping the way we perceive and leverage the vast capacities of the human mind, offering invaluable contributions to society’s overall success and collective well-being.