Imagine a team of researchers trying to crack the code of an elaborate treasure map. In this study, scientists trained and tested various types of artificial neural networks, specifically Convolutional Neural Networks (DCNNs), to understand how they perceive faces. They compared the performance of different DCNNs to human participants and found that one called VGG13 performed the best. Not only did it closely resemble human perception in terms of accuracy and visual focus, but it also showed consistent results even when presented with impaired visual inputs. This study offers valuable insights into the inner workings of artificial neural networks and highlights VGG13 as a remarkably human-like model for face perception. It opens up new avenues for studying and improving these networks by using human perception as a benchmark. So if you’re curious about the secrets behind artificial intelligence’s ability to recognize faces, dive into the fascinating research!
BackgroundConvolutional Neural Network (DCNN), with its great performance, has attracted attention of researchers from many disciplines. The studies of the DCNN and that of biological neural systems have inspired each other reciprocally. The brain-inspired neural networks not only achieve great performance but also serve as a computational model of biological neural systems.MethodsHere in this study, we trained and tested several typical DCNNs (AlexNet, VGG11, VGG13, VGG16, DenseNet, MobileNet, and EfficientNet) with a face ethnicity categorization task for experiment 1, and an emotion categorization task for experiment 2. We measured the performance of DCNNs by testing them with original and lossy visual inputs (various kinds of image occlusion) and compared their performance with human participants. Moreover, the class activation map (CAM) method allowed us to visualize the foci of the “attention” of these DCNNs.ResultsThe results suggested that the VGG13 performed the best: Its performance closely resembled human participants in terms of psychophysics measurements, it utilized similar areas of visual inputs as humans, and it had the most consistent performance with inputs having various kinds of impairments.DiscussionIn general, we examined the processing mechanism of DCNNs using a new paradigm and found that VGG13 might be the most human-like DCNN in this task. This study also highlighted a possible paradigm to study and develop DCNNs using human perception as a benchmark.
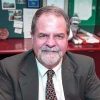
Dr. David Lowemann, M.Sc, Ph.D., is a co-founder of the Institute for the Future of Human Potential, where he leads the charge in pioneering Self-Enhancement Science for the Success of Society. With a keen interest in exploring the untapped potential of the human mind, Dr. Lowemann has dedicated his career to pushing the boundaries of human capabilities and understanding.
Armed with a Master of Science degree and a Ph.D. in his field, Dr. Lowemann has consistently been at the forefront of research and innovation, delving into ways to optimize human performance, cognition, and overall well-being. His work at the Institute revolves around a profound commitment to harnessing cutting-edge science and technology to help individuals lead more fulfilling and intelligent lives.
Dr. Lowemann’s influence extends to the educational platform BetterSmarter.me, where he shares his insights, findings, and personal development strategies with a broader audience. His ongoing mission is shaping the way we perceive and leverage the vast capacities of the human mind, offering invaluable contributions to society’s overall success and collective well-being.