Just as a detective carefully examines every piece of evidence to solve a crime, scientists are using deep learning to unravel the mysteries of noncoding genetic variants in neurodegenerative diseases. These variants, which don’t directly affect protein-coding genes, play a crucial role in the development of conditions like Alzheimer’s. To make sense of the massive amounts of complex genomic data, researchers have turned to deep learning—a cutting-edge tool that can analyze intricate patterns and relationships within these datasets. This review explores various deep learning approaches tailored for predicting how these genetic variants impact disease progression. It discusses different model architectures, development philosophies, and interpretation methods that have emerged in recent years. By understanding these variant-disease associations, researchers hope to improve patient prognosis and develop new treatment strategies. The future looks bright at the intersection of neurodegeneration, genomics, and deep learning, offering exciting opportunities to unravel the underlying mechanisms of these devastating diseases.
Determining how noncoding genetic variants contribute to neurodegenerative dementias is fundamental to understanding disease pathogenesis, improving patient prognostication, and developing new clinical treatments. Next generation sequencing technologies have produced vast amounts of genomic data on cell type-specific transcription factor binding, gene expression, and three-dimensional chromatin interactions, with the promise of providing key insights into the biological mechanisms underlying disease. However, this data is highly complex, making it challenging for researchers to interpret, assimilate, and dissect. To this end, deep learning has emerged as a powerful tool for genome analysis that can capture the intricate patterns and dependencies within these large datasets. In this review, we organize and discuss the many unique model architectures, development philosophies, and interpretation methods that have emerged in the last few years with a focus on using deep learning to predict the impact of genetic variants on disease pathogenesis. We highlight both broadly-applicable genomic deep learning methods that can be fine-tuned to disease-specific contexts as well as existing neurodegenerative disease research, with an emphasis on Alzheimer’s-specific literature. We conclude with an overview of the future of the field at the intersection of neurodegeneration, genomics, and deep learning.
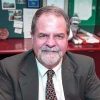
Dr. David Lowemann, M.Sc, Ph.D., is a co-founder of the Institute for the Future of Human Potential, where he leads the charge in pioneering Self-Enhancement Science for the Success of Society. With a keen interest in exploring the untapped potential of the human mind, Dr. Lowemann has dedicated his career to pushing the boundaries of human capabilities and understanding.
Armed with a Master of Science degree and a Ph.D. in his field, Dr. Lowemann has consistently been at the forefront of research and innovation, delving into ways to optimize human performance, cognition, and overall well-being. His work at the Institute revolves around a profound commitment to harnessing cutting-edge science and technology to help individuals lead more fulfilling and intelligent lives.
Dr. Lowemann’s influence extends to the educational platform BetterSmarter.me, where he shares his insights, findings, and personal development strategies with a broader audience. His ongoing mission is shaping the way we perceive and leverage the vast capacities of the human mind, offering invaluable contributions to society’s overall success and collective well-being.