Imagine trying to solve a jigsaw puzzle without knowing what the complete picture looks like. That’s what doctors face when diagnosing Alzheimer’s disease. But fear not, because scientists have come up with a brilliant solution! They’ve developed a transfer learning-based modified inception model, which is like having a puzzle-solving pro guide you through the process. This model uses deep learning and pre-processing methods to accurately diagnose Alzheimer’s disease faster than traditional methods like CT or MRI scans. It achieved an impressive accuracy of 94.92% and a sensitivity of 94.94%. That’s like acing a difficult exam! The results show that this model outperforms other state-of-the-art models in diagnosing this degenerative brain disorder. To train the model, scientists used a dataset that contained various types of brain images, including mild demented, moderate demented, and non-demented images. By continuing to explore this groundbreaking research, we can unlock even more effective tools for detecting Alzheimer’s disease early on and finding ways to slow its progression.
Alzheimer’s disease (AD) is a neurodegenerative ailment, which gradually deteriorates memory and weakens the cognitive functions and capacities of the body, such as recall and logic. To diagnose this disease, CT, MRI, PET, etc. are used. However, these methods are time-consuming and sometimes yield inaccurate results. Thus, deep learning models are utilized, which are less time-consuming and yield results with better accuracy, and could be used with ease. This article proposes a transfer learning-based modified inception model with pre-processing methods of normalization and data addition. The proposed model achieved an accuracy of 94.92 and a sensitivity of 94.94. It is concluded from the results that the proposed model performs better than other state-of-the-art models. For training purposes, a Kaggle dataset was used comprising 6,200 images, with 896 mild demented (M.D) images, 64 moderate demented (Mod.D) images, and 3,200 non-demented (N.D) images, and 1,966 veritably mild demented (V.M.D) images. These models could be employed for developing clinically useful results that are suitable to descry announcements in MRI images.
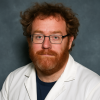
Dr. David Lowemann, M.Sc, Ph.D., is a co-founder of the Institute for the Future of Human Potential, where he leads the charge in pioneering Self-Enhancement Science for the Success of Society. With a keen interest in exploring the untapped potential of the human mind, Dr. Lowemann has dedicated his career to pushing the boundaries of human capabilities and understanding.
Armed with a Master of Science degree and a Ph.D. in his field, Dr. Lowemann has consistently been at the forefront of research and innovation, delving into ways to optimize human performance, cognition, and overall well-being. His work at the Institute revolves around a profound commitment to harnessing cutting-edge science and technology to help individuals lead more fulfilling and intelligent lives.
Dr. Lowemann’s influence extends to the educational platform BetterSmarter.me, where he shares his insights, findings, and personal development strategies with a broader audience. His ongoing mission is shaping the way we perceive and leverage the vast capacities of the human mind, offering invaluable contributions to society’s overall success and collective well-being.