Imagine you’re trying to solve a complex puzzle with pieces that constantly change shape. That’s how researchers feel when dealing with electroencephalography (EEG) signals, which are notoriously complex. But fear not! A group of clever scientists has come up with a new approach to tackle this challenge. They developed a versatile model to analyze and classify EEG signals using a combination of sparse representation measures and Swarm Intelligence techniques. By incorporating Particle Swarm Optimization, Differential Evolution, Whale Optimization Algorithm, and Backtracking Search Algorithm, they were able to make their model more adaptable. What’s even cooler is that they also employed Convolutional Neural Networks as part of their deep learning methodology. They compared their results to standard pattern recognition classifiers and the findings were remarkable. When utilizing sparse representation with deep learning, they achieved a jaw-dropping classification accuracy of 98.94%. And when implementing sparse representation with the SI-based HMM method, they still achieved an impressive accuracy of 95.70%. Talk about innovative problem-solving! If you want to dive deeper into the details of this groundbreaking study, check out the full article!
In comparison to other biomedical signals, electroencephalography (EEG) signals are quite complex in nature, so it requires a versatile model for feature extraction and classification. The structural information that prevails in the originally featured matrix is usually lost when dealing with standard feature extraction and conventional classification techniques. The main intention of this work is to propose a very novel and versatile approach for EEG signal modeling and classification. In this work, a sparse representation model along with the analysis of sparseness measures is done initially for the EEG signals and then a novel convergence of utilizing these sparse representation measures with Swarm Intelligence (SI) techniques based Hidden Markov Model (HMM) is utilized for the classification. The SI techniques utilized to compute the hidden states of the HMM are Particle Swarm Optimization (PSO), Differential Evolution (DE), Whale Optimization Algorithm (WOA), and Backtracking Search Algorithm (BSA), thereby making the HMM more pliable. Later, a deep learning methodology with the help of Convolutional Neural Network (CNN) was also developed with it and the results are compared to the standard pattern recognition classifiers. To validate the efficacy of the proposed methodology, a comprehensive experimental analysis is done over publicly available EEG datasets. The method is supported by strong statistical tests and theoretical analysis and results show that when sparse representation is implemented with deep learning, the highest classification accuracy of 98.94% is obtained and when sparse representation is implemented with SI-based HMM method, a high classification accuracy of 95.70% is obtained.
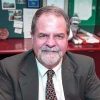
Dr. David Lowemann, M.Sc, Ph.D., is a co-founder of the Institute for the Future of Human Potential, where he leads the charge in pioneering Self-Enhancement Science for the Success of Society. With a keen interest in exploring the untapped potential of the human mind, Dr. Lowemann has dedicated his career to pushing the boundaries of human capabilities and understanding.
Armed with a Master of Science degree and a Ph.D. in his field, Dr. Lowemann has consistently been at the forefront of research and innovation, delving into ways to optimize human performance, cognition, and overall well-being. His work at the Institute revolves around a profound commitment to harnessing cutting-edge science and technology to help individuals lead more fulfilling and intelligent lives.
Dr. Lowemann’s influence extends to the educational platform BetterSmarter.me, where he shares his insights, findings, and personal development strategies with a broader audience. His ongoing mission is shaping the way we perceive and leverage the vast capacities of the human mind, offering invaluable contributions to society’s overall success and collective well-being.