Biomedical image registration is like solving a complex maze, where we need to align different images of the same structure for medical tasks. But this maze becomes even trickier when we consider the variations in intensity, texture, and anatomy caused by different imaging modalities or sample preparation methods. Enter Generative Adversarial Networks (GANs), our trusty guide in this maze! GANs have gained popularity in both mono- and cross-modal biomedical image registration because they can handle modal variance and use an adversarial training strategy. In this comprehensive survey, researchers organize GAN-based methods into four categories based on their implementation strategies: modality translation, symmetric learning, adversarial strategies, and joint training. They highlight the key concepts, contributions, advantages, and disadvantages of each strategy. Furthermore, they delve into the statistics of cited works and provide insights for future trends in GAN-based biomedical image registration studies. Ready to explore this fascinating field? Read the full article to dive into the depths of GAN-powered image registration!
Biomedical image registration refers to aligning corresponding anatomical structures among different images, which is critical to many tasks, such as brain atlas building, tumor growth monitoring, and image fusion-based medical diagnosis. However, high-throughput biomedical image registration remains challenging due to inherent variations in the intensity, texture, and anatomy resulting from different imaging modalities, different sample preparation methods, or different developmental stages of the imaged subject. Recently, Generative Adversarial Networks (GAN) have attracted increasing interest in both mono- and cross-modal biomedical image registrations due to their special ability to eliminate the modal variance and their adversarial training strategy. This paper provides a comprehensive survey of the GAN-based mono- and cross-modal biomedical image registration methods. According to the different implementation strategies, we organize the GAN-based mono- and cross-modal biomedical image registration methods into four categories: modality translation, symmetric learning, adversarial strategies, and joint training. The key concepts, the main contributions, and the advantages and disadvantages of the different strategies are summarized and discussed. Finally, we analyze the statistics of all the cited works from different points of view and reveal future trends for GAN-based biomedical image registration studies.
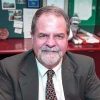
Dr. David Lowemann, M.Sc, Ph.D., is a co-founder of the Institute for the Future of Human Potential, where he leads the charge in pioneering Self-Enhancement Science for the Success of Society. With a keen interest in exploring the untapped potential of the human mind, Dr. Lowemann has dedicated his career to pushing the boundaries of human capabilities and understanding.
Armed with a Master of Science degree and a Ph.D. in his field, Dr. Lowemann has consistently been at the forefront of research and innovation, delving into ways to optimize human performance, cognition, and overall well-being. His work at the Institute revolves around a profound commitment to harnessing cutting-edge science and technology to help individuals lead more fulfilling and intelligent lives.
Dr. Lowemann’s influence extends to the educational platform BetterSmarter.me, where he shares his insights, findings, and personal development strategies with a broader audience. His ongoing mission is shaping the way we perceive and leverage the vast capacities of the human mind, offering invaluable contributions to society’s overall success and collective well-being.