Imagine you’re exploring a vast forest, trying to locate the source of different bird calls. It’s not an easy task, as the sound can bounce off trees and echo through the undergrowth. Similarly, when it comes to locating sound sources using binaural cues, traditional methods struggle with varying acoustic conditions. However, a recent study introduces a new approach using parametric embeddings – think of them as maps of sound space. These embeddings are created by a neural network that optimizes the placement of similar sound sources close together in the embedding space. The resulting structure forms a cohesive manifold, allowing for easy interpretation of the embeddings. The researchers found that this method outperforms previous unsupervised techniques and even performs comparably to direct estimation models in situations with limited training data. Additionally, they compared two learning methods and demonstrated that the embedding holds up well under both supervised and weakly supervised conditions. If you’re curious about how this new insight can help us better understand and navigate the world of binaural sound, check out the full research article!
Recent deep neural network based methods provide accurate binaural source localization performance. These data-driven models map measured binaural cues directly to source locations hence their performance highly depend on the training data distribution. In this paper, we propose a parametric embedding that maps the binaural cues to a low-dimensional space where localization can be done with a nearest-neighbor regression. We implement the embedding using a neural network, optimized to map points that are close to each other in the latent space (the space of source azimuths or elevations) to nearby points in the embedding space, thus the Euclidean distances between the embeddings reflect their source proximities, and the structure of the embeddings forms a manifold, which provides interpretability to the embeddings. We show that the proposed embedding generalizes well in various acoustic conditions (with reverberation) different from those encountered during training, and provides better performance than unsupervised embeddings previously used for binaural localization. In addition, the proposed method performs better than or equally well as a feed-forward neural network based model that directly estimates the source locations from the binaural cues, and it has better results than the feed-forward model when a small amount of training data is used. Moreover, we also compare the proposed embedding using both supervised and weakly supervised learning, and show that in both conditions, the resulting embeddings perform similarly well, but the weakly supervised embedding allows to estimate source azimuth and elevation simultaneously.
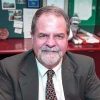
Dr. David Lowemann, M.Sc, Ph.D., is a co-founder of the Institute for the Future of Human Potential, where he leads the charge in pioneering Self-Enhancement Science for the Success of Society. With a keen interest in exploring the untapped potential of the human mind, Dr. Lowemann has dedicated his career to pushing the boundaries of human capabilities and understanding.
Armed with a Master of Science degree and a Ph.D. in his field, Dr. Lowemann has consistently been at the forefront of research and innovation, delving into ways to optimize human performance, cognition, and overall well-being. His work at the Institute revolves around a profound commitment to harnessing cutting-edge science and technology to help individuals lead more fulfilling and intelligent lives.
Dr. Lowemann’s influence extends to the educational platform BetterSmarter.me, where he shares his insights, findings, and personal development strategies with a broader audience. His ongoing mission is shaping the way we perceive and leverage the vast capacities of the human mind, offering invaluable contributions to society’s overall success and collective well-being.