Imagine your voice as a window into your body, giving doctors clues about your health. Researchers have found that analyzing speech signals can help detect early signs of Parkinson’s disease (PD), a neurodegenerative disorder. In this study, scientists focused on Mandarin language speakers and collected a large dataset to develop an automatic detection method. Unlike English, Mandarin lacks clear indicators of language disorders due to vocal organ dysfunction. To overcome this challenge, the team used machine learning algorithms to classify the articulation and phonation features in speech signals. After testing several selection models and classifiers, they achieved promising results with an accuracy of 75.76% and sensitivity of 82.44%. Interestingly, the analysis revealed that articulation features like F1, F2, DDF1, DDF2, BBE, and MFCC were more representative than phonation features. This study highlights the potential for an automatic and unobtrusive method to detect PD in Mandarin-speaking individuals. If you want to dive deeper into the research, check out the full article!
Parkinson’s disease (PD) is a neurodegenerative disorder that negatively affects millions of people. Early detection is of vital importance. As recent researches showed dysarthria level provides good indicators to the computer-assisted diagnosis and remote monitoring of patients at the early stages. It is the goal of this study to develop an automatic detection method based on newest collected Chinese dataset. Unlike English, no agreement was reached on the main features indicating language disorders due to vocal organ dysfunction. Thus, one of our approaches is to classify the speech phonation and articulation with a machine learning-based feature selection model. Based on a relatively big sample, three feature selection algorithms (LASSO, mRMR, Relief-F) were tested to select the vocal features extracted from speech signals collected in a controlled setting, followed by four classifiers (Naïve Bayes, K-Nearest Neighbor, Logistic Regression and Stochastic Gradient Descent) to detect the disorder. The proposed approach shows an accuracy of 75.76%, sensitivity of 82.44%, specificity of 73.15% and precision of 76.57%, indicating the feasibility and promising future for an automatic and unobtrusive detection on Chinese PD. The comparison among the three selection algorithms reveals that LASSO selector has the best performance regardless types of vocal features. The best detection accuracy is obtained by SGD classifier, while the best resulting sensitivity is obtained by LR classifier. More interestingly, articulation features are more representative and indicative than phonation features among all the selection and classifying algorithms. The most prominent articulation features are F1, F2, DDF1, DDF2, BBE and MFCC.
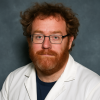
Dr. David Lowemann, M.Sc, Ph.D., is a co-founder of the Institute for the Future of Human Potential, where he leads the charge in pioneering Self-Enhancement Science for the Success of Society. With a keen interest in exploring the untapped potential of the human mind, Dr. Lowemann has dedicated his career to pushing the boundaries of human capabilities and understanding.
Armed with a Master of Science degree and a Ph.D. in his field, Dr. Lowemann has consistently been at the forefront of research and innovation, delving into ways to optimize human performance, cognition, and overall well-being. His work at the Institute revolves around a profound commitment to harnessing cutting-edge science and technology to help individuals lead more fulfilling and intelligent lives.
Dr. Lowemann’s influence extends to the educational platform BetterSmarter.me, where he shares his insights, findings, and personal development strategies with a broader audience. His ongoing mission is shaping the way we perceive and leverage the vast capacities of the human mind, offering invaluable contributions to society’s overall success and collective well-being.