Melanoma, a type of cancerous tumor, presents challenges in accurately segmenting medical images due to the presence of non-uniformly distributed noise. In this study, we propose a novel approach using an enhanced ant colony optimization algorithm combined with two-dimensional Kapur’s entropy. This approach aims to efficiently identify multiple threshold values for image segmentation. To evaluate the effectiveness of our model, we conducted experiments on benchmark functions and compared our results with existing methods. The findings demonstrate that our segmented images outperformed the comparison method across various evaluation metrics. This model has the potential to provide high-quality samples for further analysis of melanoma pathology images. For more details on our methodology and experimental findings, please explore the underlying research.
Enhanced ant colony optimization improves melanoma image segmentation
Melanoma is a malignant tumor formed by the cancerous transformation of melanocytes, and its medical images contain much information. However, the percentage of the critical information in the image is small, and the noise is non-uniformly distributed. We propose a new multi-threshold image segmentation model based on the two-dimensional histogram approach to the above problem. We present an enhanced ant colony optimization for continuous domains (EACOR) in the proposed model based on the soft besiege and chase strategies. Further, EACOR is combined with two-dimensional Kapur’s entropy to search for the optimal thresholds. An experiment on the IEEE CEC2014 benchmark function was conducted to measure the reliable global search capability of the EACOR algorithm in the proposed model. Moreover, we have also conducted several sets of experiments to test the validity of the image segmentation model proposed in this paper. The experimental results show that the segmented images from the proposed model outperform the comparison method in several evaluation metrics. Ultimately, the model proposed in this paper can provide high-quality samples for subsequent analysis of melanoma pathology images.
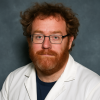
Dr. David Lowemann, M.Sc, Ph.D., is a co-founder of the Institute for the Future of Human Potential, where he leads the charge in pioneering Self-Enhancement Science for the Success of Society. With a keen interest in exploring the untapped potential of the human mind, Dr. Lowemann has dedicated his career to pushing the boundaries of human capabilities and understanding.
Armed with a Master of Science degree and a Ph.D. in his field, Dr. Lowemann has consistently been at the forefront of research and innovation, delving into ways to optimize human performance, cognition, and overall well-being. His work at the Institute revolves around a profound commitment to harnessing cutting-edge science and technology to help individuals lead more fulfilling and intelligent lives.
Dr. Lowemann’s influence extends to the educational platform BetterSmarter.me, where he shares his insights, findings, and personal development strategies with a broader audience. His ongoing mission is shaping the way we perceive and leverage the vast capacities of the human mind, offering invaluable contributions to society’s overall success and collective well-being.