Imagine having the ability to recognize objects without any prior experience or training. Well, it turns out that deep neural networks can do just that! In a recent study, scientists used a model neural network to investigate how object detection can emerge spontaneously in untrained networks. They found that even before any visual training, certain units in the network showed a strong preference for specific object classes and remained consistent in their recognition despite various transformations like rotation or scaling. This innate capability allowed the untrained network to perform object-detection tasks accurately, even with heavily modified images. The researchers also discovered that the invariant object tuning resulted from random connections between non-invariant units in the network. These findings may explain how our own brains achieve object detection and provide valuable insights for improving artificial intelligence systems. To delve deeper into this fascinating research, check out the full article!
The ability to perceive visual objects with various types of transformations, such as rotation, translation, and scaling, is crucial for consistent object recognition. In machine learning, invariant object detection for a network is often implemented by augmentation with a massive number of training images, but the mechanism of invariant object detection in biological brains—how invariance arises initially and whether it requires visual experience—remains elusive. Here, using a model neural network of the hierarchical visual pathway of the brain, we show that invariance of object detection can emerge spontaneously in the complete absence of learning. First, we found that units selective to a particular object class arise in randomly initialized networks even before visual training. Intriguingly, these units show robust tuning to images of each object class under a wide range of image transformation types, such as viewpoint rotation. We confirmed that this “innate” invariance of object selectivity enables untrained networks to perform an object-detection task robustly, even with images that have been significantly modulated. Our computational model predicts that invariant object tuning originates from combinations of non-invariant units via random feedforward projections, and we confirmed that the predicted profile of feedforward projections is observed in untrained networks. Our results suggest that invariance of object detection is an innate characteristic that can emerge spontaneously in random feedforward networks.
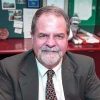
Dr. David Lowemann, M.Sc, Ph.D., is a co-founder of the Institute for the Future of Human Potential, where he leads the charge in pioneering Self-Enhancement Science for the Success of Society. With a keen interest in exploring the untapped potential of the human mind, Dr. Lowemann has dedicated his career to pushing the boundaries of human capabilities and understanding.
Armed with a Master of Science degree and a Ph.D. in his field, Dr. Lowemann has consistently been at the forefront of research and innovation, delving into ways to optimize human performance, cognition, and overall well-being. His work at the Institute revolves around a profound commitment to harnessing cutting-edge science and technology to help individuals lead more fulfilling and intelligent lives.
Dr. Lowemann’s influence extends to the educational platform BetterSmarter.me, where he shares his insights, findings, and personal development strategies with a broader audience. His ongoing mission is shaping the way we perceive and leverage the vast capacities of the human mind, offering invaluable contributions to society’s overall success and collective well-being.