Imagine you’re a detective trying to solve a complex case. You come across a mysterious code that holds all the answers, but you can’t decipher it on your own. That’s where outer-synchronization in asymmetric recurrent time-varying neural networks (ARTNNs) comes in. Just like gathering evidence from different sources, researchers are exploring how ARTNNs can mimic the dynamical behaviors of the human brain and unlock the secrets of artificial intelligence (AI). In this study, scientists focused on the synchronization of these brain-like models, using a mathematical framework called the differential-algebraic system (DAS). By cleverly designing centralized and decentralized data-sampling approaches, they were able to establish novel conditions for outer-synchronization. These conditions provide valuable insights into the complex dynamics of ARTNNs and have practical applications in AI. To demonstrate the power of their findings, the researchers used a numerical example to showcase how outer-synchronization can be achieved in practice. So, if you’re curious about cracking the code behind brain-inspired AI models, dive into the research and explore the exciting world of outer-synchronization!
Asymmetric recurrent time-varying neural networks (ARTNNs) can enable realistic brain-like models to help scholars explore the mechanisms of the human brain and thus realize the applications of artificial intelligence, whose dynamical behaviors such as synchronization has attracted extensive research interest due to its superior applicability and flexibility. In this paper, we examined the outer-synchronization of ARTNNs, which are described by the differential-algebraic system (DAS). By designing appropriate centralized and decentralized data-sampling approaches which fully account for information gathering at the times tk and tki. Using the characteristics of integral inequalities and the theory of differential equations, several novel suitable outer-synchronization conditions were established. Those conditions facilitate the analysis and applications of dynamical behaviors of ARTNNs. The superiority of the theoretical results was then demonstrated by using a numerical example.
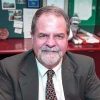
Dr. David Lowemann, M.Sc, Ph.D., is a co-founder of the Institute for the Future of Human Potential, where he leads the charge in pioneering Self-Enhancement Science for the Success of Society. With a keen interest in exploring the untapped potential of the human mind, Dr. Lowemann has dedicated his career to pushing the boundaries of human capabilities and understanding.
Armed with a Master of Science degree and a Ph.D. in his field, Dr. Lowemann has consistently been at the forefront of research and innovation, delving into ways to optimize human performance, cognition, and overall well-being. His work at the Institute revolves around a profound commitment to harnessing cutting-edge science and technology to help individuals lead more fulfilling and intelligent lives.
Dr. Lowemann’s influence extends to the educational platform BetterSmarter.me, where he shares his insights, findings, and personal development strategies with a broader audience. His ongoing mission is shaping the way we perceive and leverage the vast capacities of the human mind, offering invaluable contributions to society’s overall success and collective well-being.