Imagine you’re building a machine brain, and you want it to learn like a human brain. Turns out, the brain-inspired approach of predictive coding can significantly improve the performance of challenging tasks for machines! In the world of artificial neural networks, backpropagation has been the star algorithm for training. But critics argue that it doesn’t align with how our brains work. So, can we simulate the brain’s learning mechanism to boost machine learning in difficult tasks? That’s what this study set out to discover. Instead of using backpropagation, they used predictive coding—a more biologically plausible learning algorithm. The researchers conducted multiple experiments on tasks where machines struggle, such as incremental learning, long-tailed recognition, and few-shot recognition. And guess what? The results were outstanding! Predictive coding not only reduced catastrophic forgetting during incremental learning but also addressed classification bias in long-tailed recognition. Moreover, it showed great promise in accurately predicting targets with only a few samples. By drawing parallels between predictive coding networks and the human brain, the study sheds light on their potential in general machine learning. If you’re excited about this revolutionary twist, dive into the research to learn more!
Brain-inspired Predictive Coding Improves the Performance of Machine Challenging Tasks
Backpropagation has been regarded as the most favorable algorithm for training artificial neural networks. However, it has been criticized for its biological implausibility because its learning mechanism contradicts the human brain. Although backpropagation has achieved super-human performance in various machine learning applications, it often shows limited performance in specific tasks. We collectively referred to such tasks as machine-challenging tasks (MCTs) and aimed to investigate methods to enhance machine learning for MCTs. Specifically, we start with a natural question: Can a learning mechanism that mimics the human brain lead to the improvement of MCT performances? We hypothesized that a learning mechanism replicating the human brain is effective for tasks where machine intelligence is difficult. Multiple experiments corresponding to specific types of MCTs where machine intelligence has room to improve performance were performed using predictive coding, a more biologically plausible learning algorithm than backpropagation. This study regarded incremental learning, long-tailed, and few-shot recognition as representative MCTs. With extensive experiments, we examined the effectiveness of predictive coding that robustly outperformed backpropagation-trained networks for the MCTs. We demonstrated that predictive coding-based incremental learning alleviates the effect of catastrophic forgetting. Next, predictive coding-based learning mitigates the classification bias in long-tailed recognition. Finally, we verified that the network trained with predictive coding could correctly predict corresponding targets with few samples. We analyzed the experimental result by drawing analogies between the properties of predictive coding networks and those of the human brain and discussing the potential of predictive coding networks in general machine learning.
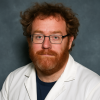
Dr. David Lowemann, M.Sc, Ph.D., is a co-founder of the Institute for the Future of Human Potential, where he leads the charge in pioneering Self-Enhancement Science for the Success of Society. With a keen interest in exploring the untapped potential of the human mind, Dr. Lowemann has dedicated his career to pushing the boundaries of human capabilities and understanding.
Armed with a Master of Science degree and a Ph.D. in his field, Dr. Lowemann has consistently been at the forefront of research and innovation, delving into ways to optimize human performance, cognition, and overall well-being. His work at the Institute revolves around a profound commitment to harnessing cutting-edge science and technology to help individuals lead more fulfilling and intelligent lives.
Dr. Lowemann’s influence extends to the educational platform BetterSmarter.me, where he shares his insights, findings, and personal development strategies with a broader audience. His ongoing mission is shaping the way we perceive and leverage the vast capacities of the human mind, offering invaluable contributions to society’s overall success and collective well-being.