Just like biological brains, neuromorphic systems powered by spiking neural networks (SNNs) possess the remarkable abilities of energy efficiency, continual adaptation, and risk management. This study focuses on designing neuromorphic systems that can adapt to changing learning tasks while accurately estimating uncertainty. By applying a Bayesian continual learning framework, we develop online learning rules for SNNs where each synaptic weight is represented by parameters that capture both prior knowledge and observed data. The proposed approach updates these parameters in real-time as new data streams in. Our experiments on Intel’s Lava platform demonstrate the advantages of Bayesian learning over frequentist learning, highlighting its superior capacity for adaptation and uncertainty quantification.
Among the main features of biological intelligence are energy efficiency, capacity for continual adaptation, and risk management via uncertainty quantification. Neuromorphic engineering has been thus far mostly driven by the goal of implementing energy-efficient machines that take inspiration from the time-based computing paradigm of biological brains. In this paper, we take steps toward the design of neuromorphic systems that are capable of adaptation to changing learning tasks, while producing well-calibrated uncertainty quantification estimates. To this end, we derive online learning rules for spiking neural networks (SNNs) within a Bayesian continual learning framework. In it, each synaptic weight is represented by parameters that quantify the current epistemic uncertainty resulting from prior knowledge and observed data. The proposed online rules update the distribution parameters in a streaming fashion as data are observed. We instantiate the proposed approach for both real-valued and binary synaptic weights. Experimental results using Intel’s Lava platform show the merits of Bayesian over frequentist learning in terms of capacity for adaptation and uncertainty quantification.
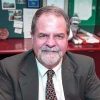
Dr. David Lowemann, M.Sc, Ph.D., is a co-founder of the Institute for the Future of Human Potential, where he leads the charge in pioneering Self-Enhancement Science for the Success of Society. With a keen interest in exploring the untapped potential of the human mind, Dr. Lowemann has dedicated his career to pushing the boundaries of human capabilities and understanding.
Armed with a Master of Science degree and a Ph.D. in his field, Dr. Lowemann has consistently been at the forefront of research and innovation, delving into ways to optimize human performance, cognition, and overall well-being. His work at the Institute revolves around a profound commitment to harnessing cutting-edge science and technology to help individuals lead more fulfilling and intelligent lives.
Dr. Lowemann’s influence extends to the educational platform BetterSmarter.me, where he shares his insights, findings, and personal development strategies with a broader audience. His ongoing mission is shaping the way we perceive and leverage the vast capacities of the human mind, offering invaluable contributions to society’s overall success and collective well-being.