Just like how an artist evaluates the visual appeal of their artwork, scientists are now using an interpretable approach to automatically assess the aesthetics of remote sensing images. Remote sensing images, which are commonly used in fields like environmental protection and education, have not received much attention in terms of aesthetic evaluation. To bridge this gap, researchers used a convolutional neural network (CNN), a type of deep learning model that mimics the human neural system, to assess the aesthetic quality of these images. They created the Remote Sensing Aesthetics Dataset (RSAD) by collecting remote sensing images from Google Earth and labeling them based on expert photographers’ judgments on color harmony, light and shadow, prominent theme, and visual balance. The researchers then trained a ResNet-18 architecture using RSAD and found that their method accurately identifies visually pleasing remote sensing images. To further enhance interpretability, they used an algorithm called Gradient-weighted Class Activation Mapping (Grad-CAM) to highlight the important areas in the images that influenced the model’s decision. This research opens up new possibilities for using remote sensing images in non-scientific applications such as art and contributes to our understanding of how deep learning models can evaluate image aesthetics. If you’re curious about how artificial intelligence can judge the beauty of satellite images, check out the full article!
The increase of remote sensing images in recent decades has resulted in their use in non-scientific fields such as environmental protection, education, and art. In this situation, we need to focus on the aesthetic assessment of remote sensing, which has received little attention in research. While according to studies on human brain’s attention mechanism, certain areas of an image can trigger visual stimuli during aesthetic evaluation. Inspired by this, we used convolutional neural network (CNN), a deep learning model resembling the human neural system, for image aesthetic assessment. So we propose an interpretable approach for automatic aesthetic assessment of remote sensing images. Firstly, we created the Remote Sensing Aesthetics Dataset (RSAD). We collected remote sensing images from Google Earth, designed the four evaluation criteria of remote sensing image aesthetic quality—color harmony, light and shadow, prominent theme, and visual balance—and then labeled the samples based on expert photographers’ judgment on the four evaluation criteria. Secondly, we feed RSAD into the ResNet-18 architecture for training. Experimental results show that the proposed method can accurately identify visually pleasing remote sensing images. Finally, we provided a visual explanation of aesthetic assessment by adopting Gradient-weighted Class Activation Mapping (Grad-CAM) to highlight the important image area that influenced model’s decision. Overall, this paper is the first to propose and realize automatic aesthetic assessment of remote sensing images, contributing to the non-scientific applications of remote sensing and demonstrating the interpretability of deep-learning based image aesthetic evaluation.
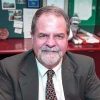
Dr. David Lowemann, M.Sc, Ph.D., is a co-founder of the Institute for the Future of Human Potential, where he leads the charge in pioneering Self-Enhancement Science for the Success of Society. With a keen interest in exploring the untapped potential of the human mind, Dr. Lowemann has dedicated his career to pushing the boundaries of human capabilities and understanding.
Armed with a Master of Science degree and a Ph.D. in his field, Dr. Lowemann has consistently been at the forefront of research and innovation, delving into ways to optimize human performance, cognition, and overall well-being. His work at the Institute revolves around a profound commitment to harnessing cutting-edge science and technology to help individuals lead more fulfilling and intelligent lives.
Dr. Lowemann’s influence extends to the educational platform BetterSmarter.me, where he shares his insights, findings, and personal development strategies with a broader audience. His ongoing mission is shaping the way we perceive and leverage the vast capacities of the human mind, offering invaluable contributions to society’s overall success and collective well-being.