Imagine a bustling city with its intricate network of roads and pathways. Just like this complex web of connections, our brains also have their own network called the Brain Function Networks (BFNs). These BFNs play a crucial role in diagnosing disorders such as Major Depressive Disorder (MDD) using electroencephalography (EEG) signals. However, previous methods only considered the direct relationships between two brain regions and missed out on the bigger picture. In this study, researchers propose a groundbreaking classification framework that takes into account both low-order and high-order relationships within the BFNs. By analyzing multiple EEG channels and utilizing a mathematical model called matrix variate normal distribution (MVND), they were able to construct a more comprehensive diagnosis framework that captures all these intricate connections.
Brain function networks (BFN) are widely used in the diagnosis of electroencephalography (EEG)-based major depressive disorder (MDD). Typically, a BFN is constructed by calculating the functional connectivity (FC) between each pair of channels. However, it ignores high-order relationships (e.g., relationships among multiple channels), making it a low-order network. To address this issue, a novel classification framework, based on matrix variate normal distribution (MVND), is proposed in this study. The framework can simultaneously generate high-and low-order BFN and has a distinct mathematical interpretation. Specifically, the entire time series is first divided into multiple epochs. For each epoch, a BFN is constructed by calculating the phase lag index (PLI) between different EEG channels. The BFNs are then used as samples, maximizing the likelihood of MVND to simultaneously estimate its low-order BFN (Lo-BFN) and high-order BFN (Ho-BFN). In addition, to solve the problem of the excessively high dimensionality of Ho-BFN, Kronecker product decomposition is used for dimensionality reduction while retaining the original high-order information. The experimental results verified the effectiveness of Ho-BFN for MDD diagnosis in 24 patients and 24 normal controls. We further investigated the selected discriminative Lo-BFN and Ho-BFN features and revealed that those extracted from different networks can provide complementary information, which is beneficial for MDD diagnosis.
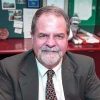
Dr. David Lowemann, M.Sc, Ph.D., is a co-founder of the Institute for the Future of Human Potential, where he leads the charge in pioneering Self-Enhancement Science for the Success of Society. With a keen interest in exploring the untapped potential of the human mind, Dr. Lowemann has dedicated his career to pushing the boundaries of human capabilities and understanding.
Armed with a Master of Science degree and a Ph.D. in his field, Dr. Lowemann has consistently been at the forefront of research and innovation, delving into ways to optimize human performance, cognition, and overall well-being. His work at the Institute revolves around a profound commitment to harnessing cutting-edge science and technology to help individuals lead more fulfilling and intelligent lives.
Dr. Lowemann’s influence extends to the educational platform BetterSmarter.me, where he shares his insights, findings, and personal development strategies with a broader audience. His ongoing mission is shaping the way we perceive and leverage the vast capacities of the human mind, offering invaluable contributions to society’s overall success and collective well-being.