Imagine you’re a DJ trying to mix the perfect track. You want to find the right balance of beats and melodies that will get everyone on their feet. Similar to this, scientists are trying to optimize spiking neural models of the striatum, a brain structure involved in action selection. They want to understand how the brain solves this complex problem. But tuning these models is no easy task! It requires a lot of expert knowledge and computational power. That’s where black-box and surrogate optimization come in. These methods act like music mixers, helping scientists find the right configuration of their models. In this study, researchers tested different optimization methods, including SurrogateOpt, RBFOpt, and DIRECT-GL, along with a random search. SurrogateOpt emerged as the best option, finding high-quality configurations faster than an expert while RBFOpt and the random search came in second. DIRECT-GL, unfortunately, lagged behind. This study shows that with the right optimization techniques, scientists can fine-tune their brain models more efficiently and autonomously. So if you’re curious about how they did it, check out the full research article!
The basal ganglia (BG) is a brain structure that has long been proposed to play an essential role in action selection, and theoretical models of spiking neurons have tried to explain how the BG solves this problem. A recently proposed functional and biologically inspired network model of the striatum (an important nucleus of the BG) is based on spike-timing-dependent eligibility (STDE) and captured important experimental features of this nucleus. The model can recognize complex input patterns and consistently choose rewarded actions to respond to such sensory inputs. However, model tuning is challenging due to two main reasons. The first is the expert knowledge required, resulting in tedious and potentially biased trial-and-error procedures. The second is the computational cost of assessing model configurations (approximately 1.78 h per evaluation). This study addresses the model tuning problem through numerical optimization. Considering the cost of assessing solutions, the selected methods stand out due to their low requirements for solution evaluations and compatibility with high-performance computing. They are the SurrogateOpt solver of Matlab and the RBFOpt library, both based on radial basis function approximations, and DIRECT-GL, an enhanced version of the widespread black-box optimizer DIRECT. Besides, a parallel random search serves as a baseline reference of the outcome of opting for sophisticated methods. SurrogateOpt turns out to be the best option for tuning this kind of model. It outperforms, on average, the quality of the configuration found by an expert and works significantly faster and autonomously. RBFOpt and the random search share the second position, but their average results are below the option found by hand. Finally, DIRECT-GL follows this line becoming the worst-performing method.
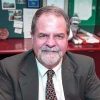
Dr. David Lowemann, M.Sc, Ph.D., is a co-founder of the Institute for the Future of Human Potential, where he leads the charge in pioneering Self-Enhancement Science for the Success of Society. With a keen interest in exploring the untapped potential of the human mind, Dr. Lowemann has dedicated his career to pushing the boundaries of human capabilities and understanding.
Armed with a Master of Science degree and a Ph.D. in his field, Dr. Lowemann has consistently been at the forefront of research and innovation, delving into ways to optimize human performance, cognition, and overall well-being. His work at the Institute revolves around a profound commitment to harnessing cutting-edge science and technology to help individuals lead more fulfilling and intelligent lives.
Dr. Lowemann’s influence extends to the educational platform BetterSmarter.me, where he shares his insights, findings, and personal development strategies with a broader audience. His ongoing mission is shaping the way we perceive and leverage the vast capacities of the human mind, offering invaluable contributions to society’s overall success and collective well-being.