Imagine a Brain-Computer Interface (BCI) as a powerful controller that allows you to seamlessly navigate through the world, much like a skilled gamer maneuvering through a virtual reality landscape. Scientists have been working hard to improve the performance of BCIs, and they have made significant progress by using an innovative approach. By analyzing temporal and spectral features from electroencephalography (EEG) signals and applying deep learning neural networks to classify these features, they have achieved remarkable results in predicting the motor actions that a person imagines. They developed an algorithm that combines time-based and frequency-based features, resulting in an average performance increase of 3.50% compared to existing state-of-the-art methods. Their algorithm achieves an impressive accuracy of 90.08% on one dataset and 88.74% on another dataset, outperforming the average benchmarks. To further enhance BCI performance, the researchers suggest incorporating multiple modalities and utilizing neural network classification protocols for a variety of tasks. This exciting research opens up possibilities for enhancing BCIs and expanding their applications in empowering individuals with limited mobility or enabling more immersive man-machine interactions. Dive into the fascinating details of this study to gain a deeper understanding of the potential of BCIs!
Brain-Computer Interfaces (BCIs) are increasingly useful for control. Such BCIs can be used to assist individuals who lost mobility or control over their limbs, for recreational purposes such as gaming or semi-autonomous driving, or as an interface toward man-machine integration. Thus far, the performance of algorithms used for thought decoding has been limited. We show that by extracting temporal and spectral features from electroencephalography (EEG) signals and, following, using deep learning neural network to classify those features, one can significantly improve the performance of BCIs in predicting which motor action was imagined by a subject. Our movement prediction algorithm uses Sequential Backward Selection technique to jointly choose temporal and spectral features and a radial basis function neural network for the classification. The method shows an average performance increase of 3.50% compared to state-of-the-art benchmark algorithms. Using two popular public datasets our algorithm reaches 90.08% accuracy (compared to an average benchmark of 79.99%) on the first dataset and 88.74% (average benchmark: 82.01%) on the second dataset. Given the high variability within- and across-subjects in EEG-based action decoding, we suggest that using features from multiple modalities along with neural network classification protocol is likely to increase the performance of BCIs across various tasks.
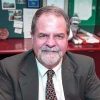
Dr. David Lowemann, M.Sc, Ph.D., is a co-founder of the Institute for the Future of Human Potential, where he leads the charge in pioneering Self-Enhancement Science for the Success of Society. With a keen interest in exploring the untapped potential of the human mind, Dr. Lowemann has dedicated his career to pushing the boundaries of human capabilities and understanding.
Armed with a Master of Science degree and a Ph.D. in his field, Dr. Lowemann has consistently been at the forefront of research and innovation, delving into ways to optimize human performance, cognition, and overall well-being. His work at the Institute revolves around a profound commitment to harnessing cutting-edge science and technology to help individuals lead more fulfilling and intelligent lives.
Dr. Lowemann’s influence extends to the educational platform BetterSmarter.me, where he shares his insights, findings, and personal development strategies with a broader audience. His ongoing mission is shaping the way we perceive and leverage the vast capacities of the human mind, offering invaluable contributions to society’s overall success and collective well-being.