Imagine you’re teaching a friend how to bake cookies, but you only have a few ingredients and not enough chocolate chips . You know that your friend learned how to bake a cake before, so you decide to transfer the knowledge from that experience to the new recipe. In a similar way, scientists are using transfer learning to diagnose Alzheimer’s disease (AD) more accurately by leveraging knowledge from a related dataset. This study introduces a transfer learning framework called TrGB, based on the gradient boosting machine (GBM). TrGB assigns weights to instances based on their distribution similarities or differences with the target data. By doing so, it transfers valuable information while avoiding negative influence. The results of the experiments using ADNI as the source domain and Mount Sinai dataset as the target showed that TrGB improved classification accuracy significantly in comparison to conventional methods. For instance, TrGB increased accuracy by 1.5% and 4.5% for CN vs. MCI and multiclass classification, respectively. By transferring knowledge from the CN vs. AD classification, it also boosted early MCI vs. late MCI classification by 5%. So, if you’re curious about how transfer learning can enhance Alzheimer’s diagnosis using machine learning algorithms, check out the research!
Early detection of Alzheimer’s disease (AD) during the Mild Cognitive Impairment (MCI) stage could enable effective intervention to slow down disease progression. Computer-aided diagnosis of AD relies on a sufficient amount of biomarker data. When this requirement is not fulfilled, transfer learning can be used to transfer knowledge from a source domain with more amount of labeled data than available in the desired target domain. In this study, an instance-based transfer learning framework is presented based on the gradient boosting machine (GBM). In GBM, a sequence of base learners is built, and each learner focuses on the errors (residuals) of the previous learner. In our transfer learning version of GBM (TrGB), a weighting mechanism based on the residuals of the base learners is defined for the source instances. Consequently, instances with different distribution than the target data will have a lower impact on the target learner. The proposed weighting scheme aims to transfer as much information as possible from the source domain while avoiding negative transfer. The target data in this study was obtained from the Mount Sinai dataset which is collected and processed in a collaborative 5-year project at the Mount Sinai Medical Center. The Alzheimer’s Disease Neuroimaging Initiative (ADNI) dataset was used as the source domain. The experimental results showed that the proposed TrGB algorithm could improve the classification accuracy by 1.5 and 4.5% for CN vs. MCI and multiclass classification, respectively, as compared to the conventional methods. Also, using the TrGB model and transferred knowledge from the CN vs. AD classification of the source domain, the average score of early MCI vs. late MCI classification improved by 5%.
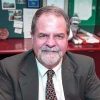
Dr. David Lowemann, M.Sc, Ph.D., is a co-founder of the Institute for the Future of Human Potential, where he leads the charge in pioneering Self-Enhancement Science for the Success of Society. With a keen interest in exploring the untapped potential of the human mind, Dr. Lowemann has dedicated his career to pushing the boundaries of human capabilities and understanding.
Armed with a Master of Science degree and a Ph.D. in his field, Dr. Lowemann has consistently been at the forefront of research and innovation, delving into ways to optimize human performance, cognition, and overall well-being. His work at the Institute revolves around a profound commitment to harnessing cutting-edge science and technology to help individuals lead more fulfilling and intelligent lives.
Dr. Lowemann’s influence extends to the educational platform BetterSmarter.me, where he shares his insights, findings, and personal development strategies with a broader audience. His ongoing mission is shaping the way we perceive and leverage the vast capacities of the human mind, offering invaluable contributions to society’s overall success and collective well-being.