Imagine your heart as a traffic controller, directing the flow of life-giving oxygenated blood to every corner of your body. But sometimes, there’s a roadblock – clogged or constricted arteries that restrict the flow. Detecting heart disease accurately is crucial, and that’s where this exciting research comes in! Scientists have developed a cutting-edge heart disease diagnosis application that harnesses the power of IoT sensor data and deep learning algorithms. Just like a skilled investigator, this intelligent application analyzes data collected from real heart patients through IoT sensors, clearing away any noise using the K-means technique. It then gets to work extracting valuable features and employing a mathematical tool called Linear Quadratic Discriminant Analysis. Finally, the powerful Deep Graph Convolutional Network (DG_ConvoNet) steps in to classify and predict heart diseases. And guess what? This extraordinary system achieves an impressive accuracy of 96%! If you’re itching to learn more about this groundbreaking research and its potential implications for future healthcare, don’t wait – dive into the details now!
Heart disease is an emerging health issue in the medical field, according to WHO every year around 10 billion people are affected with heart abnormalities. Arteries in the heart generate oxygenated blood to all body parts, however sometimes blood vessels become clogged or restrained due to cardiac issues. Past heart diagnosis applications are outdated and suffer from poor performance. Therefore, an intelligent heart disease diagnosis application design is required. In this research work, internet of things (IoT) sensor data with a deep learning-based heart diagnosis application is designed. The heart disease IoT sensor data is collected from the University of California Irvine machine learning repository free open-source dataset which is useful for training the deep graph convolutional network (DG_ConvoNet) deep learning network. The testing data has been collected from the Cleveland Clinic Foundation; it is a collection of 350 real-time clinical instances from heart patients through IoT sensors. The K-means technique is employed to remove noise in sensor data and clustered the unstructured data. The features are extracted to employ Linear Quadratic Discriminant Analysis. DG_ConvoNet is a deep learning process to classify and predict heart diseases. The diagnostic application achieves an accuracy of 96%, sensitivity of 80%, specificity of 73%, precision of 90%, F-Score of 79%, and area under the ROC curve of 75% implementing the proposed model.
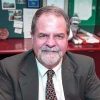
Dr. David Lowemann, M.Sc, Ph.D., is a co-founder of the Institute for the Future of Human Potential, where he leads the charge in pioneering Self-Enhancement Science for the Success of Society. With a keen interest in exploring the untapped potential of the human mind, Dr. Lowemann has dedicated his career to pushing the boundaries of human capabilities and understanding.
Armed with a Master of Science degree and a Ph.D. in his field, Dr. Lowemann has consistently been at the forefront of research and innovation, delving into ways to optimize human performance, cognition, and overall well-being. His work at the Institute revolves around a profound commitment to harnessing cutting-edge science and technology to help individuals lead more fulfilling and intelligent lives.
Dr. Lowemann’s influence extends to the educational platform BetterSmarter.me, where he shares his insights, findings, and personal development strategies with a broader audience. His ongoing mission is shaping the way we perceive and leverage the vast capacities of the human mind, offering invaluable contributions to society’s overall success and collective well-being.