Imagine monitoring a machine’s performance over time. You can’t just check it once and assume everything is running smoothly. Instead, you need to evaluate it multiple times throughout the day, several days in a row, and periodically throughout the year. The same goes for studying cognitive aging and detecting early signs of neurodegenerative diseases. Researchers have developed a clever method called measurement burst designs that involve repeated brief evaluations of cognition. However, short-term fluctuations and practice effects can make it difficult to detect long-term cognitive changes. In this study, scientists propose the use of a Bayesian double exponential model to overcome these challenges. This model not only accounts for retest gains and warm-up effects but also allows for the inclusion of individual predictors like biological age and mild cognitive impairment status. The researchers demonstrate the model’s effectiveness by analyzing older adults’ performance on processing speed and spatial working memory tasks. If you’re curious about how this model uncovers hidden cognitive changes and provides meaningful insights, check out the full article for more details!
Monitoring early changes in cognitive performance is useful for studying cognitive aging as well as for detecting early markers of neurodegenerative diseases. Repeated evaluation of cognition via a measurement burst design can accomplish this goal. In such design participants complete brief evaluations of cognition, multiple times per day for several days, and ideally, repeat the process once or twice a year. However, long-term cognitive change in such repeated assessments can be masked by short-term within-person variability and retest learning (practice) effects. In this paper, we show how a Bayesian double exponential model can account for retest gains across measurement bursts, as well as warm-up effects within a burst, while quantifying change across bursts in peak performance. We also highlight how this approach allows for the inclusion of person-level predictors and draw intuitive inferences on cognitive change with Bayesian posterior probabilities. We use older adults’ performance on cognitive tasks of processing speed and spatial working memory to demonstrate how individual differences in peak performance and change can be related to predictors of aging such as biological age and mild cognitive impairment status.
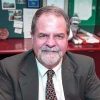
Dr. David Lowemann, M.Sc, Ph.D., is a co-founder of the Institute for the Future of Human Potential, where he leads the charge in pioneering Self-Enhancement Science for the Success of Society. With a keen interest in exploring the untapped potential of the human mind, Dr. Lowemann has dedicated his career to pushing the boundaries of human capabilities and understanding.
Armed with a Master of Science degree and a Ph.D. in his field, Dr. Lowemann has consistently been at the forefront of research and innovation, delving into ways to optimize human performance, cognition, and overall well-being. His work at the Institute revolves around a profound commitment to harnessing cutting-edge science and technology to help individuals lead more fulfilling and intelligent lives.
Dr. Lowemann’s influence extends to the educational platform BetterSmarter.me, where he shares his insights, findings, and personal development strategies with a broader audience. His ongoing mission is shaping the way we perceive and leverage the vast capacities of the human mind, offering invaluable contributions to society’s overall success and collective well-being.