Imagine you’re trying to perfect your swing in baseball. You’ve tried different training methods, but there’s always room for improvement. That’s exactly what scientists are doing with spiking neuronal networks (SNNs) when it comes to motor control. While artificial neural networks (ANNs) have been successful in learning sensory-motor behaviors, SNNs have lagged behind. But fear not! Researchers have taken on the challenge by exploring different learning mechanisms to boost SNN performance.
They trained SNNs to tackle the CartPole reinforcement learning (RL) control problem using two learning mechanisms: spike-timing-dependent reinforcement learning (STDP-RL) and evolutionary strategy (EVOL). STDP-RL mimics how the brain learns through reinforcing connections based on timing, while EVOL fits model neural responsiveness to recordings. By comparing the results, they discovered that EVOL is a powerful method for training SNNs in sensory-motor behaviors!
This study not only enhances our understanding of SNNs but also provides insights into the multi-timescale learning mechanisms and dynamics of neuronal circuits. It’s like uncovering a secret recipe for improving your swing in baseball! Curious about these groundbreaking findings? Dive into the research and embrace the exciting world of SNNs in motor control!
Artificial neural networks (ANNs) have been successfully trained to perform a wide range of sensory-motor behaviors. In contrast, the performance of spiking neuronal network (SNN) models trained to perform similar behaviors remains relatively suboptimal. In this work, we aimed to push the field of SNNs forward by exploring the potential of different learning mechanisms to achieve optimal performance. We trained SNNs to solve the CartPole reinforcement learning (RL) control problem using two learning mechanisms operating at different timescales: (1) spike-timing-dependent reinforcement learning (STDP-RL) and (2) evolutionary strategy (EVOL). Though the role of STDP-RL in biological systems is well established, several other mechanisms, though not fully understood, work in concert during learning in vivo. Recreating accurate models that capture the interaction of STDP-RL with these diverse learning mechanisms is extremely difficult. EVOL is an alternative method and has been successfully used in many studies to fit model neural responsiveness to electrophysiological recordings and, in some cases, for classification problems. One advantage of EVOL is that it may not need to capture all interacting components of synaptic plasticity and thus provides a better alternative to STDP-RL. Here, we compared the performance of each algorithm after training, which revealed EVOL as a powerful method for training SNNs to perform sensory-motor behaviors. Our modeling opens up new capabilities for SNNs in RL and could serve as a testbed for neurobiologists aiming to understand multi-timescale learning mechanisms and dynamics in neuronal circuits.
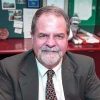
Dr. David Lowemann, M.Sc, Ph.D., is a co-founder of the Institute for the Future of Human Potential, where he leads the charge in pioneering Self-Enhancement Science for the Success of Society. With a keen interest in exploring the untapped potential of the human mind, Dr. Lowemann has dedicated his career to pushing the boundaries of human capabilities and understanding.
Armed with a Master of Science degree and a Ph.D. in his field, Dr. Lowemann has consistently been at the forefront of research and innovation, delving into ways to optimize human performance, cognition, and overall well-being. His work at the Institute revolves around a profound commitment to harnessing cutting-edge science and technology to help individuals lead more fulfilling and intelligent lives.
Dr. Lowemann’s influence extends to the educational platform BetterSmarter.me, where he shares his insights, findings, and personal development strategies with a broader audience. His ongoing mission is shaping the way we perceive and leverage the vast capacities of the human mind, offering invaluable contributions to society’s overall success and collective well-being.