Imagine trying to solve a complex puzzle with only a few scattered pieces. That’s how researchers have felt about diagnosing Autism Spectrum Disorder (ASD) – a condition that affects 1% of the population. But now, with the help of structural magnetic resonance imaging (sMRI) and machine learning (ML), they are making promising strides in understanding ASD. Like detectives studying fingerprints at a crime scene, scientists use sMRI to analyze the volumetric and geometric features of the autistic brain. However, inconsistent findings have hindered progress in this field. ML, particularly deep learning (DL), has emerged as a powerful tool in disease diagnosis, including ASD. By training ML algorithms on large datasets of brain scans, researchers are starting to uncover reliable biomarkers for ASD. While challenges still remain, such as limited sample sizes, ongoing research shows great potential in revolutionizing ASD diagnostic methods.The advancements in ML are bringing us closer to a future where clinicians can detect ASD more accurately and provide tailored treatment plans. Curious? Dive into the underlying research to learn more!
Autism spectrum disorder (ASD) is a complex neurodevelopmental disorder that affects approximately 1% of the population and causes significant burdens. ASD’s pathogenesis remains elusive; hence, diagnosis is based on a constellation of behaviors. Structural magnetic resonance imaging (sMRI) studies have shown several abnormalities in volumetric and geometric features of the autistic brain. However, inconsistent findings prevented most contributions from being translated into clinical practice. Establishing reliable biomarkers for ASD using sMRI is crucial for the correct diagnosis and treatment. In recent years, machine learning (ML) and specifically deep learning (DL) have quickly extended to almost every sector, notably in disease diagnosis. Thus, this has led to a shift and improvement in ASD diagnostic methods, fulfilling most clinical diagnostic requirements. However, ASD discovery remains difficult. This review examines the ML-based ASD diagnosis literature over the past 5 years. A literature-based taxonomy of the research landscape has been mapped, and the major aspects of this topic have been covered. First, we provide an overview of ML’s general classification pipeline and the features of sMRI. Next, representative studies are highlighted and discussed in detail with respect to methods, and biomarkers. Finally, we highlight many common challenges and make recommendations for future directions. In short, the limited sample size was the main obstacle; Thus, comprehensive data sets and rigorous methods are necessary to check the generalizability of the results. ML technologies are expected to advance significantly in the coming years, contributing to the diagnosis of ASD and helping clinicians soon.
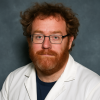
Dr. David Lowemann, M.Sc, Ph.D., is a co-founder of the Institute for the Future of Human Potential, where he leads the charge in pioneering Self-Enhancement Science for the Success of Society. With a keen interest in exploring the untapped potential of the human mind, Dr. Lowemann has dedicated his career to pushing the boundaries of human capabilities and understanding.
Armed with a Master of Science degree and a Ph.D. in his field, Dr. Lowemann has consistently been at the forefront of research and innovation, delving into ways to optimize human performance, cognition, and overall well-being. His work at the Institute revolves around a profound commitment to harnessing cutting-edge science and technology to help individuals lead more fulfilling and intelligent lives.
Dr. Lowemann’s influence extends to the educational platform BetterSmarter.me, where he shares his insights, findings, and personal development strategies with a broader audience. His ongoing mission is shaping the way we perceive and leverage the vast capacities of the human mind, offering invaluable contributions to society’s overall success and collective well-being.