Imagine flipping through old photo albums with your grandparents, and just by observing their brain activity, you can tell how they’re feeling. A team of scientists has developed a groundbreaking method that uses Electroencephalogram (EEG) signals to recognize emotions in older adults. Like analyzing muscle artifacts during speech or using fewer electrodes for better comfort, this technology measures emotional states accurately. The proposed CTA-CNN-Bi-LSTM framework utilizes eight specific channels in the brain to encode EEG data, extracting spatial information and learning bi-directional temporal patterns for robust emotion recognition. In fact, this innovative framework surpasses earlier methods and achieves an impressive 98.75% average recognition accuracy for positive, negative, and neutral emotions. By merging cutting-edge neural networks with a keen understanding of older adults’ emotional well-being, this research fosters connections between generations and enhances the mental health of our elder loved ones. To dive deeper into the study, check out the full article!
Reminiscence and conversation between older adults and younger volunteers using past photographs are very effective in improving the emotional state of older adults and alleviating depression. However, we need to evaluate the emotional state of the older adult while conversing on the past photographs. While Electroencephalogram (EEG) has a significantly stronger association with emotion than other physiological signals, the challenge is to eliminate muscle artifacts in the EEG during speech as well as to reduce the number of dry electrodes to improve user comfort while maintaining high emotion recognition accuracy. Therefore, we proposed the CTA-CNN-Bi-LSTM emotion recognition framework. EEG signals of 8 channels (P3, P4, F3, F4, F7, F8, T7, and T8) were first implemented in the MEMD-CCA method on three brain regions separately (Frontal, Temporal, Parietal) to remove the muscle artifacts then were fed into the Channel-Temporal attention module to get the weights of channels and temporal points most relevant to the positive, negative and neutral emotions to recode the EEG data. A Convolutional Neural Networks (CNNs) module then extracted the spatial information in the new EEG data to obtain the spatial feature maps which were then sequentially inputted into a Bi-LSTM module to learn the bi-directional temporal information for emotion recognition. Finally, we designed four group experiments to demonstrate that the proposed CTA-CNN-Bi-LSTM framework outperforms the previous works. And the highest average recognition accuracy of the positive, negative, and neutral emotions achieved 98.75%.
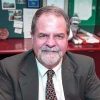
Dr. David Lowemann, M.Sc, Ph.D., is a co-founder of the Institute for the Future of Human Potential, where he leads the charge in pioneering Self-Enhancement Science for the Success of Society. With a keen interest in exploring the untapped potential of the human mind, Dr. Lowemann has dedicated his career to pushing the boundaries of human capabilities and understanding.
Armed with a Master of Science degree and a Ph.D. in his field, Dr. Lowemann has consistently been at the forefront of research and innovation, delving into ways to optimize human performance, cognition, and overall well-being. His work at the Institute revolves around a profound commitment to harnessing cutting-edge science and technology to help individuals lead more fulfilling and intelligent lives.
Dr. Lowemann’s influence extends to the educational platform BetterSmarter.me, where he shares his insights, findings, and personal development strategies with a broader audience. His ongoing mission is shaping the way we perceive and leverage the vast capacities of the human mind, offering invaluable contributions to society’s overall success and collective well-being.